 |
 |
 |
 |
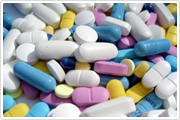
The Saccharomyces cerevisiae (yeast) genome has approximately 6200 known genes. Large-scale experiments have shown approximately 73% (~4500) of the genes to be non-essential - a yeast missing any single non-essential gene is still viable. Of course, viability is not guaranteed when multiple non-essential genes are knocked-out. In fact, the study of double-knockouts (yeast with two disabled genes) can provide key insights into the functional relationships between genes. For example, if a double-knockout of a pair of non-essential genes X and Y is nonviable, it might suggest that X and Y are elements of redundant or parallel biological pathways. In this arrangement, either X or Y can fill the same essential role; when both are disabled, the cell will not survive. This is only one, among many, functional explanations for this synthetic lethal phenomenon.
The collaborative yeast group at the University of Toronto, led by Charlie Boone's lab, has developed an automated method for the systematic construction of double-knockouts. These knockouts form the basis of Synthetic Genetic Array (SGA) analysis. A number of local groups (including those of Brenda Andrews, Mike Tyers, and Andrew Emili) have been using SGA experimental data to infer functional relationships among genes. In collaboration with Andrew Emili's lab we are interested in exploring the use of SGA data to create a platform for high-throughput drug screening.
For the Non-Biologist: Genes play important roles in cell function. Over the past twenty years, molecular biologists have developed techniques for experimentally manipulating genes to gain insight into their function. One manipulation of particular interest is the knockout experiment where a target gene is essentially turned off. In this work we are utilizing the results of experiments where pairs of genes are turned off. By studying these altered yeast we can gain insights into gene function. In our work, we are utilizing the experimental results of knockout pairs (also known as double-knockouts) to assist in identifying new pharmacological compounds.
Students: Izhar Wallach, Gabe Musso
Collaborators: Andrew Emili (CCBR, Dept. of Medical Genetics)
|
 |
 |
 |
 |
 |
 |
 |
 |

The collaborative yeast group at the University of Toronto is hard at work completing viability studies on the approximately 8 million yeast double-knockouts (4,000 x 4,000). Using the currently available partial data we have developed a proof-of-concept result demonstrating the utility of SGA data for rapidly and efficiently identifying ligands capable of targeting (and inhibiting) non-essential yeast genes.
Idea: When represented as a matrix, the SGA data matrix S contains elements S(i,j) representing the viability of the double-knockout of gene i and gene j. We consider each row of the SGA data matrix to be a knockout profile, q (the binary viability pattern) of a single gene g. Casting the SGA data into a Machine Learning framework, the knockout profile for each gene is a binary feature vector for that gene (i.e., the knockout profile represents the viability pattern of an organism missing gene g). Now, consider a ligand binding experiment where a single ligand is screened against n single-gene knockouts (i.e., one knockout for each of the n non-essential genes). The result of this experiment is a knockout profile of the ligand. Intuitively, this experiment is similar to the SGA experiments. In both cases, each feature of a knockout profile corresponds to the synthetic lethality of two non-essential yeast genes i and j. In a traditional SGA experiment, each gene is knocked out at the genetic level. In the ligand screen, one gene is knocked out at the genetic level while the second gene’s product is inhibited by the ligand. If we assume that an active drug will target only a single gene product, then a drug’s knockout profile should match a single gene’s knockout profile. Identifying the gene whose knockout profile matches that of the ligand identifies a potential target of ligand binding (figure right).
Our method employs a reduction of the problem to Set Cover for which we identify an optimal solution using Integer Linear Programming. Although our approach does not explicitly seek to uniquely identify the molecular target of binding ligands, we demonstrate that in the majority of cases we are able to identify a unique target. Our findings suggest that once complete SGA data becomes available our approach will form the basis of a novel platform for high-throughput ligand screening.
Rather than collecting an entire ligand's knockout profile (which would require ~4000 experiments) we are looking to identify a minimal (yet informative) subset of features (single gene knockouts) capable of forming a generic screen for ligand binding. Rather than screening against 4000 single gene knockouts, we hope to identify a subset of several hundred genes which will provide the same amount of information.
Thus far, we have identified a set of 78 features, corresponding to 78 single gene knockout experiments, capable of identifying ligand binding to any of 892 non-essential yeast genes. Compared to the naive approach of screening against the complete set of 4500 single gene knockouts, our results represent a 50-fold reduction in the number of wet-lab experiments. Furthermore, in the vast majority of cases we are able to uniquely identify the active ligand’s gene target. We are now working closely with Andrew Emili's lab to carry these results into the wetlab!

|
 |
 |
 |
 |
|
|