 |
 |
 |
 |
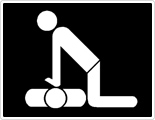
When closed-chest cardiac massage was first described it was seen as a method to resuscitate patients in good physiological condition who were "the victim of acute insult, whether it be from drowning, electrical shock, untoward effect of drugs, anesthetic accident, heart block, acute MI, or surgery". The new method drastically improved the outcome of cardiac arrests. Over time, CPR evolved from unorganized actions by untrained staff to efficient and synchronized teamwork by trained personnel, and became a fundamental part of medical care offered to all patients in cardiac arrest. However, due to its use on more critically ill patients, survival from CPR to hospital discharge declined from 24% in 1961 to 14% in 1981. In the 1990s, responding to demands for patient autonomy and participation in decision making, many US hospitals began instituting "Do Not Resuscitate" (DNR) policies allowing patients or their families to determine that no resuscitation be attempted in the event of a cardiac arrest. Despite thoughtful and well-written policies regarding DNR orders, less than 25% of seriously ill patients discuss preferences for cardiopulmonary resuscitation with their physicians; furthermore, less than half of in-patients who prefer not to receive CPR actually have DNR orders written. The biggest obstacle to the DNR discussion is physician uncertainty in prognosticating about CPR outcomes. Physicians simply feel they can't provide patients with useful information. Accurate prediction of the outcomes of in-hospital cardiopulmonary resuscitation would be helpful to patients and their physicians in deciding whether to forego this intervention.
Unfortunately, the few existing tools in this area of code survival prognostication are either out-dated, too complicated, or rely heavily on post-arrest data (i.e., type of arrhythmia, time down, etc.) to be helpful in the code status discussion. Therefore, a clinical prediction rule, utilizing pre-arrest data would empower physicians to prognosticate more accurately thereby increasing the frequency of code status discussions and promoting patient autonomy.
Students: Izhar Wallach, Satyam Mirja
Collaborators: Dr. Hilary Ryder (Internal Medicine, DHMC)
|
 |
 |
 |
 |
 |
 |
 |
 |

We are working with Dr. Hilary Ryder, an internal-medicine physician at the Dartmouth-Hitchcock Medical Center (DHMC) to develop a clinical prediction tool for predicting CPR outcomes. More generally, we are developing a suite of tools for generating integer-based weight-constrained linear classifiers. These classifiers form the basis of most clinical prediction rules.
Dr. Ryder's group retrospectively reviewed medical and nursing records of all adult in-patient CPR attempts at DHMC over a three year period. Patients were identified retrospectively from the CPR committee log of in-hospital cardiopulmonary arrests. Cardiac arrest was defined "the cessation of cardiac mechanical activity, confirmed by the absence of a detectable pulse, unresponsiveness, and apnea". The study cohort consisted of all consecutive patients aged 18 years and older with an in-patient cardiac arrest and an attempted resuscitation by the resuscitation team. All consecutive patients arresting in during data collection were eligible for the study. Syncope, seizures, and primary respiratory arrest were excluded as causes of arrest. Patients whose resuscitation began outside of the hospital were excluded. Approximately 400 eligible patients were identified.
We used admission variables (defined as values obtained within 24 hours of admission) because in our experience conversations about CPR typically take place on admission, when only this data is available. We pre-specified all features used in the prognostic model by first developing a list of general features that were expected to be available for all patients and had been identified in the literature as varying significantly between survivors and non-survivors. This process identified approximately 40 features falling into five broad categories: demographic variables, functional status, medical diagnoses, physical findings, and laboratory values. With the goal of minimizing false negatives (i.e., patients predicted not to survive CPR should not have survived), we constructed an integer-based linear classifier with eleven features (a compromise between predictive accuracy and the expected size of a clinical prediction rule). We developed a modification of Linear Discriminant Analysis (LDA) and used the method to identify a clinical prediction rule with 11 features. Our preliminary clinical prediction rule (Dartmouth Score) has a sensitivity of 97%, a positive predictive value (PPV) of 32%, and a negative predictive value (NPV) of 95%. These results are consistent with our goal of identifying all patients who might conceivably survive to discharge (the high sensitivity and NPV). This compares favorably with previously published methods (PAM, PAR, MPI) computed on our same validation set (table below). This work was presented at the 30th annual meeting of the Society of General Internal Medicine (2007).
|
Prediction Rule |
Sens. |
PPV |
NPV |
|
|
Dartmouth Score |
97% |
32% |
95% |
|
|
PAM |
75% |
37% |
84% |
|
|
PAR |
75% |
28% |
73% |
|
|
MPI |
75% |
41% |
81% |
|
|
We are currently working on several extensions.
Developing a General Integer-Based Weight-Constrained Linear Classifier: We are formalizing the mathematical framework of our new classification scheme. We are writing a paper and will be preparing a software distribution for general use in the summer of 2008.
Completing the Dartmouth Score: We are completing the development of the Dartmouth Score for predicting CPR outcomes. Our original dataset has been augmented with additional patients and we are rerunning our analysis. In hopes of making our clinical prediction rule available to the medical community we are preparing it for publication.
Seeking Collaborations: Are you a clinician with patient data looking to build a clinical prediction rule? Please send us an email!

|
 |
 |
 |
 |
|
|