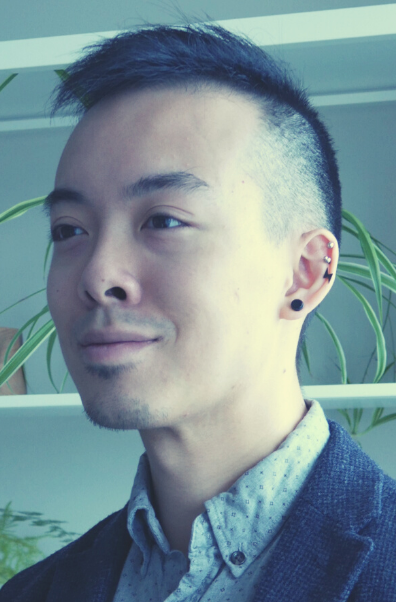
Behavioral science and HCI through massive datasets.
I do research on social systems by measuring how user behaviors and attitudes are tied to desirable outcomes. My work falls under computational social science and uses a variety of approaches from behavioral data science (e.g. user modeling and survey science), applied ML/NLP (e.g. embeddings), and HCI (e.g. experiments and user studies).
Cognitive Biases in LLMs
We show that LLMs like GPT can mistrust beneficial algorithmic advice through 2 experiments with 5 real-world datasets.
Unfair Risk Assessments
We ran an online experiment simulating credit risk assessments and found that people judge an unfair algorithmic assessor more harshly than an identically unfair human agent.
Politicians in Social Media
We analyze millions of news articles on Reddit to show that coverage of major politicians is more selectively-consumed and affectively polarized on social media.
Polarized Online News
We investigate millions of links shared on Reddit to show that polarized news is restricted to but endemic in a small group of hyper-partisan communities.
Cross-Timezone Work
We combined a trace of millions of meetings, a survey, and interviews to quantify how difficult but diversifying it is for temporally-distant collaborators to meet.
Diverse Consumption
We modeled billions of listening events at Spotify to show that people seek different kinds of content variety over their lifecycles.
Screentime and Well-being
We combinatorially searched over millions of regressions to robustly measure how time spent online is tied to user well-being.