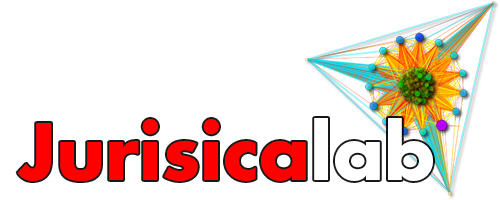
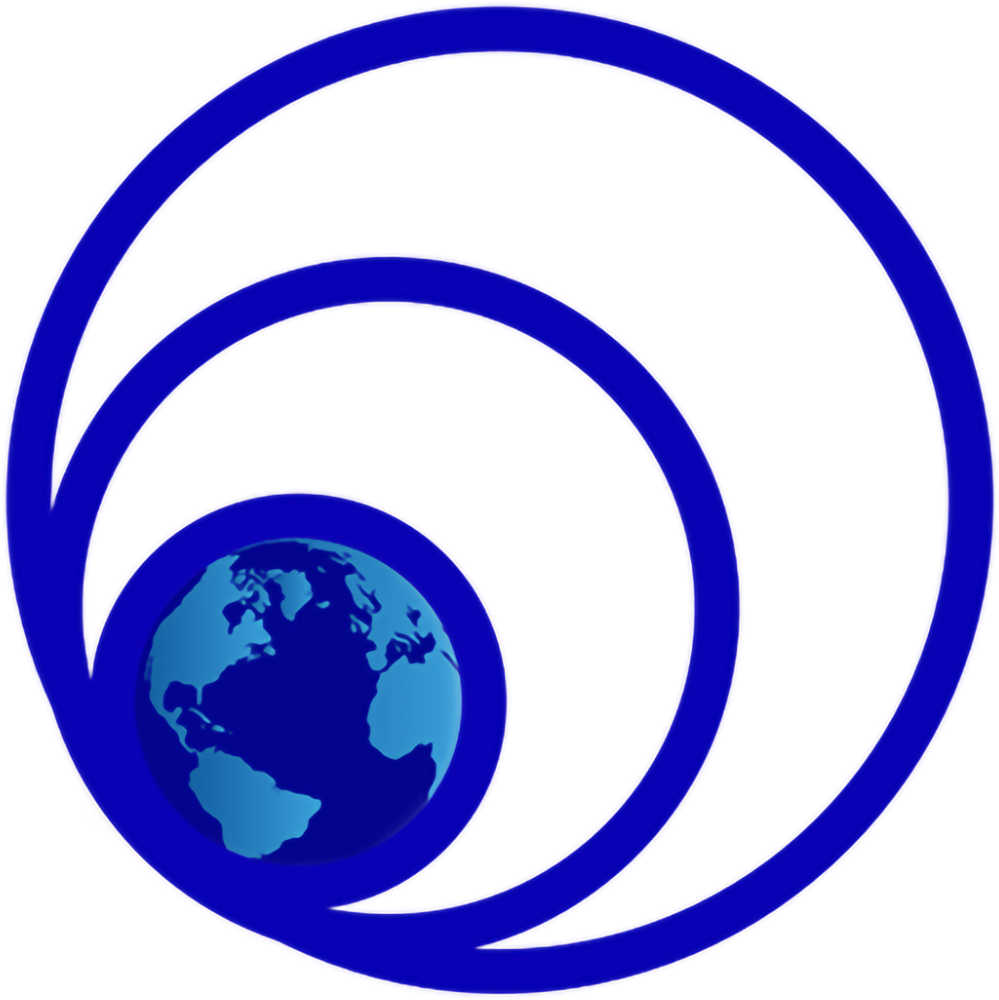
World Community Grid is a public, high-performance computing platform available for "open science/open data" research that benefits humanity. WCG has been created and funded by a philanthropic initiative of IBM Corporate Social Responsibility since 2004 till 2021, to empower anyone with a computer, Android or Raspberry Pi device to contribute to research on health and sustainability. Check out our Youtube Channel, where you can find videos such as; How World Community Grid works and How can World Community Grid transform scientific research.
WCG embraces open-source and open-data sharing policy. The policy contributes to collaborative and reproducible science. Access to data is a significant factor driving science today. So far WCG has generated over a petabyte of data, available freely to the global research community. Our scientific and contributing partners include: Scripps Research, Harvard University, The University of Texas Medical Branch, Pierre et Marie Curie University, University of Washington, Chiba Cancer Center Research Institute, University of Cape Town, UMDNJ-Robert Wood Johnson Medical School and The Cancer Institute of New Jersey, Institute for Systems Biology, New York University, Georgia Tech and many others.
WCG meets important security standards. Following security by design principles, the physical servers are located within a managed services environment which follows the Compute Canada security standards, and communication with client-side machines are secured using private digital keys.
WCG uses Berkeley Open Infrastructure for Network Computing (BOINC) clients, built at U Berkeley, and tested by over 4 million volunteers since 1995. BOINC is used world-wide by over 45 projects, including: ERN (LHC@Home), University of Washington (Rosetta@Home), Max Planck Institute for Graviational Physics - Hanover (Einstein@Home), Rensselaer Polytechnic Institute (Milkyway@Home), Oxford University (ClimatePrediction.net).
We strive to make the world a better and healthier place for all of humanity. Our mission is to accelerate science by creating a supercomputer empowered by a global community of volunteers. Ultimately, our volunteers and partners have helped create an impressive global - yet individually driven - people's supercomputer!
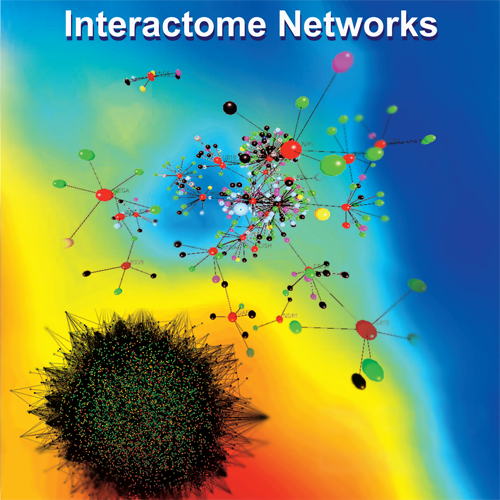
Data portals and algorithms have been instrumental in many clinical and preclinical studies, including those in arthritis (e.g., PMID: 38267619, 37894979, 37532285, 35791923, 36474475, 35321117, 35157043, 34803912, 34669443, 34588660, 33303908, 32738291, 36741392), brain research (e.g., PMID: 38350995, 35852739, 35172120, 34897024, 32357304), cancer (e.g., PMID: 37478172, 34881186, 34072436, 33142239, 32443704, 32015424, 31980649, 31780666, 30922393, 36815540, 30912767, 30796022, 30659026, 30222135, 29507679, 29483644, 28766011, 28174235, 27956147), immunology (e.g., PMID: 38444851, 35264564, 34645905, 34255742, 36597912, 36238301), and transplant (e.g., PMID: 38446901, 34557585, 34033948, 33863738, 32900843, 32453760, 30801542).
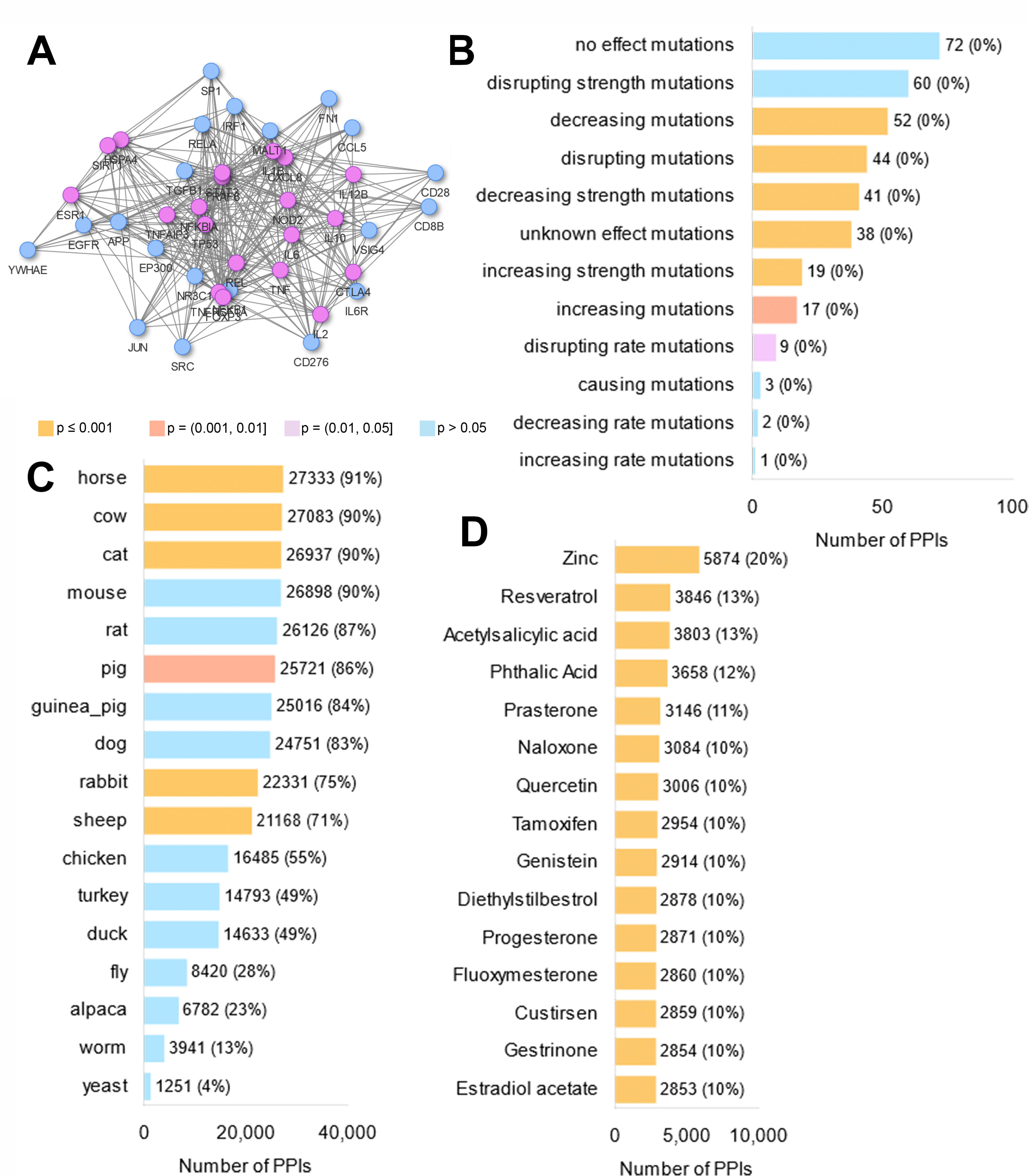
IID is the first database providing tissue-specific protein-protein interactions (PPIs) for model organisms (yeast, worm, fly, rat, mouse) and human, providing access to 1,421,199 human protein interactions covering 20,147 proteins, annotated with 133 tissues and 92 diseases (PMID: 34755877, 34902122, 33262342, 30407591, 29220074). IID was expanded through the collaboration with high-throughput biologists, application to tissue-specific and disease-specific context (e.g., PMID: 35156780, 34606829, 34881186, 32415080, 32357304, 31980649, 31780666, 28766011, 28298427, 28065597, 27956147), and as part of international IMEx consortium of PPI curated databases (e.g., PMID: 33262342, 33206959, 30602777, 29642841). These analyses led to tissue- and disease-specific models and validated insights (e.g., PMID: 28766011, 31780666, 30912767, 32357304, 30659026).
IID is an active member of IMEx consortium, which has been included in the Global Biodata Coalition (GBC) in 2023. Discontinuation of GBC resources would have a highly detrimental impact on the global research endeavour; and there are only 52 such critical biodata resources worldwide.
Kotlyar M, Pastrello C, Ahmed Z, Chee J, Varyova Z, Jurisica I, IID 2021: Towards context-specific protein interaction analyses by increased coverage, enhanced annotation and enrichment analysis. Nucl Acids Res, 50(D1):D640-D647, 2022.Kotlyar, M., Pastrello, C., Malik, Z., Jurisica, I., IID 2018 update: context-specific physical protein-protein interactions in human, model organisms and domesticated species. Nucl Acids Res, 47(D1):D581-D589, 2019.
Kotlyar M, Pastrello C, Sheahan N, Jurisica I. Integrated interactions database: tissue-specific view of the human and model organism interactomes. Nucl Acids Res, 44(D1):D536-41, 2016.
Kotlyar, M, Wong, SWH, Pastrello, C, Jurisica, I. Improving Analysis and Annotation of Microarray Data with Protein Interactions. Ed. G. Agapito. Microarray Data Analysis, Methods Mol Biol, vol. 2401: 51-68, 2022.
Pastrello C, Kotlyar M, Jurisica I., Informed Use of Protein-Protein Interaction Data: A Focus on the Integrated Interactions Database (IID). Methods Mol Biol., 2074:125-134, 2020.
Hauschild, A-C, Pastrello, C, Kotlyar, M and Jurisica, I. Protein-protein interaction data, their quality, and major public databases. Ed. N. Przulj. Analyzing Network Data in Biology and Medicine, An Interdisciplinary Textbook for Biological, Medical and Computational Scientists, Cambridge University Press, Cambridge, UK, pp.151-192, 2019.
Go to IID home page
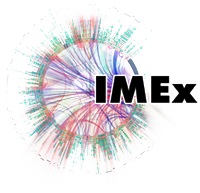
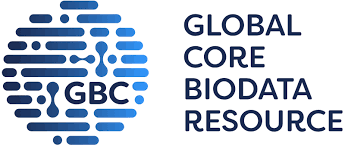
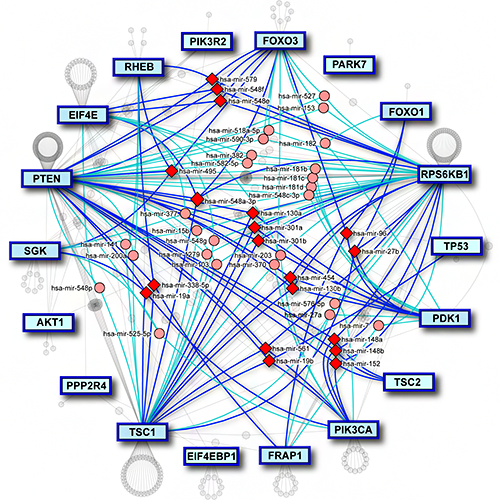
MirDIP 5 has also extended content and functionality by associating contexts with microRNAs, genes, and microRNA-gene interactions. It includes curated microRNA and gene expression data from 20 resources and information on 330 tissue and disease contexts for 2,657 microRNAs, 27,576 genes and 123,651,910 gene-microRNA-tissue interactions. MirDIP 5 has also improved usability by enabling the user to search the database using precursor IDs. miRAnno (PMID: 34297061) is now integrated into mirDIP, which enables a network-based identification of pathways linked to specific microRNAs. MirDIP API facilitates access to integrated predictions. In addition, users can now analyze their results using Drugst.One, where a drug-gene network is created using only the user’s genes in a specific pathway.
Houschild AC, Pastrello C, Ekaputeri GKA, Bethune-Waddell D, Abovsky M, Ahmed Z, Kotlyar M Lu R, Jurisica I. MirDIP 5.2: tissue context annotation and novel microRNA curation, Nuncl Acids Res, 51(D1):D217-D225, 2023.
Tokar T, Pastrello C, Abovsky M, Rahmati S, Jurisica I. miRAnno—network-based functional microRNA annotation, Bioinformatics, 38(2):592–593, 2022.
Tokar, T., Pastrello, C., Rossos, A., Abovsky, M., Hauschild, A.C., Tsay, M., Lu, R., Jurisica, I. mirDIP 4.1 – Integrative database of human microRNA target predictions, Nucl Acids Res, 46(D1): D360-D370, 2018.
Shirdel EA, Xie W, Mak TW, Jurisica I. NAViGaTing the micronome - using multiple microRNA prediction databases to identify signalling pathway-associated microRNAs. PLoS One. 6(2):e17429, 2011.
Go to mirDIP home page
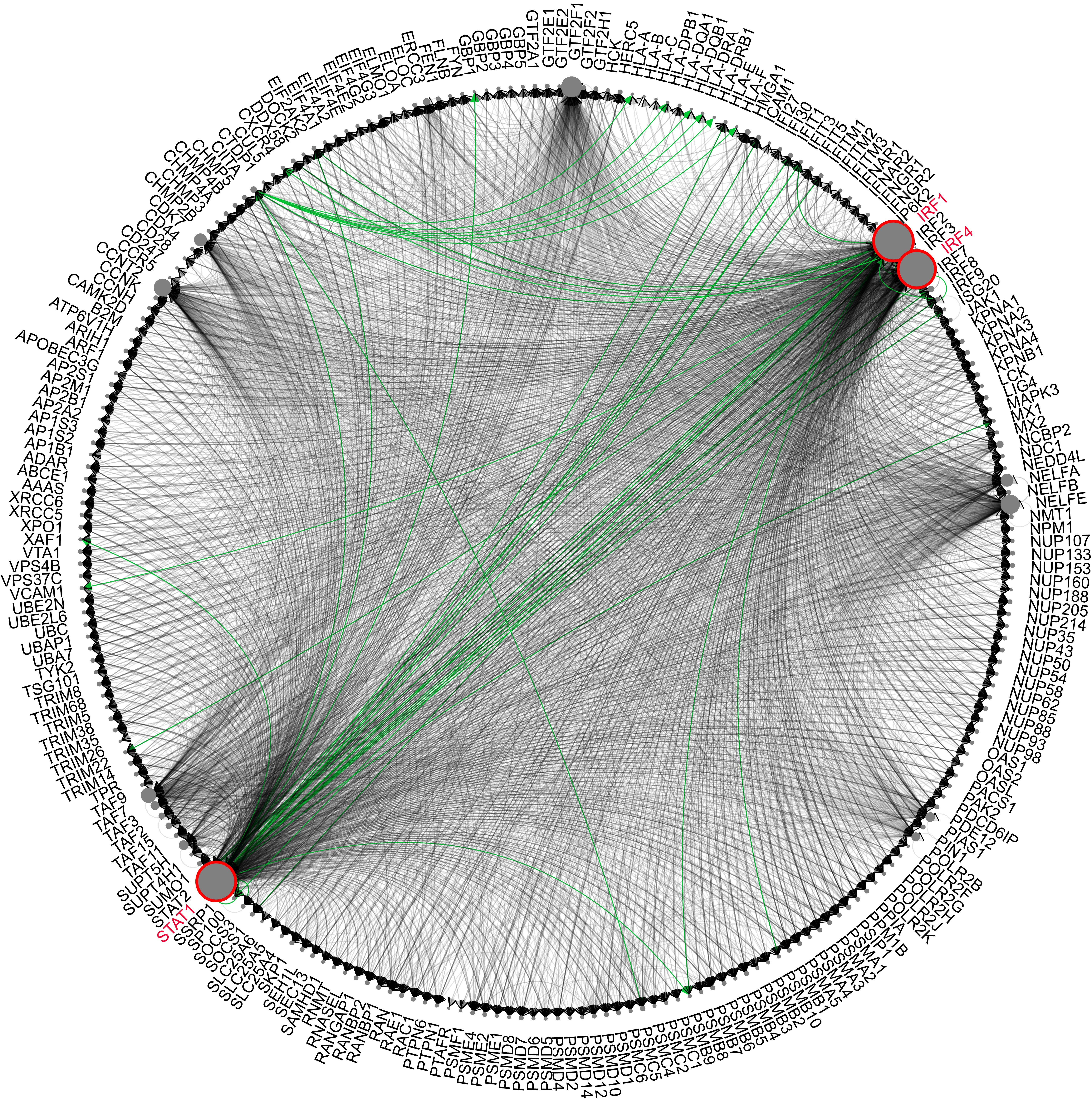
Catrin integrates ~13.2 million unique transcription factor:target gene associations, involving 2,871 putative transcription factors and covering nearly 25 thousand genes. In addition to the web interface, Catrin provides programmatic access via API, to maximize utilization of this database.
Endisha H, Datta P, Sharma A, Nakamura S, Rossomacha E, Younan C, Ali SA, Tavallaee G, Lively S, Potla P, Shestopaloff K, Rockel JS, Krawetz R, Mahomed NN, Jurisica I, Gandhi R, Kapoor M. MicroRNA-34a-5p Promotes Joint Destruction During Osteoarthritis. Arthritis Rheumatol. 2021 Mar;73(3):426-439. doi: 10.1002/art.41552. Epub 2021 Feb 8. PMID: 33034147; PMCID: PMC7986901.
Tavallaee G, Lively S, Rockel JS, Ali SA, Im M, Sarda C, Mitchell GM, Rossomacha E, Nakamura S, Potla P, Gabrial S, Matelski J, Ratneswaran A, Perry K, Hinz B, Gandhi R, Jurisica I, Kapoor M. Contribution of MicroRNA-27b-3p to Synovial Fibrotic Responses in Knee Osteoarthritis. Arthritis Rheumatol. 2022 Dec;74(12):1928-1942. doi: 10.1002/art.42285. Epub 2022 Nov 11. PMID: 35791923; PMCID: PMC10946865.
França TT, Al-Sbiei A, Bashir G, Mohamed YA, Salgado RC, Barreiros LA, Maria da Silva Napoleão S, Weber CW, Fernandes Severo Ferreira J, Aranda CS, Prando C, de Barros Dorna MB, Jurisica I, Fernandez-Cabezudo MJ, Ochs HD, Condino-Neto A, Al-Ramadi BK, Cabral-Marques O. CD40L modulates transcriptional signatures of neutrophils in the bone marrow associated with development and trafficking. JCI Insight. 2021 Aug 23;6(16):e148652. doi: 10.1172/jci.insight.148652. PMID: 34255742; PMCID: PMC8410015.
Chakraborty M, Chu K, Shrestha A, Revelo XS, Zhang X, Gold MJ, Khan S, Lee M, Huang C, Akbari M, Barrow F, Chan YT, Lei H, Kotoulas NK, Jovel J, Pastrello C, Kotlyar M, Goh C, Michelakis E, Clemente-Casares X, Ohashi PS, Engleman EG, Winer S, Jurisica I, Tsai S, Winer DA. Mechanical Stiffness Controls Dendritic Cell Metabolism and Function. Cell Rep. 2021 Jan 12;34(2):108609. doi: 10.1016/j.celrep.2020.108609. PMID: 33440149.
D'Angelo E, Pastrello C, Biccari A, Marangio A, Sensi F, Crotti S, Fassan M, Jurisica I, Pucciarelli S, Agostini M. An integrated multiomics analysis of rectal cancer patients identified POU2F3 as a putative druggable target and entinostat as a cytotoxic enhancer of 5-fluorouracil. Int J Cancer. 2023 Jul 15;153(2):437-449. doi: 10.1002/ijc.34478. Epub 2023 Mar 23. PMID: 36815540.
McEvoy CM, Clotet-Freixas S, Tokar T, Pastrello C, Reid S, Batruch I, RaoPeters AAE, Kaths JM, Urbanellis P, Farkona S, Van JAD, Urquhart BL, John R, Jurisica I, Robinson LA, Selzner M, Konvalinka A. Normothermic Ex-vivo Kidney Perfusion in a Porcine Auto-Transplantation Model Preserves the Expression of Key Mitochondrial Proteins: An Unbiased Proteomics Analysis. Mol Cell Proteomics. 2021;20:100101. doi: 10.1016/j.mcpro.2021.100101. Epub 2021 May 23. PMID: 34033948; PMCID: PMC8253910.
Clotet-Freixas S, Zaslaver O, Kotlyar M, Pastrello C, Quaile AT, McEvoy CM, Saha AD, Farkona S, Boshart A, Zorcic K, Neupane S, Manion K, Allen M, Chan M, Chen X, Arnold AP, Sekula P, Steinbrenner I, Köttgen A, Dart AB, Wicklow B, McGavock JM, Blydt-Hansen TD, Barrios C, Riera M, Soler MJ, Isenbrandt A, Lamontagne-Proulx J, Pradeloux S, Coulombe K, Soulet D, Rajasekar S, Zhang B, John R, Mehrotra A, Gehring A, Puhka M, Jurisica I, Woo M, Scholey JW, Röst H, Konvalinka A. Sex differences in kidney metabolism may reflect sex-dependent outcomes in human diabetic kidney disease. Sci Transl Med. 2024 Mar 6;16(737):eabm2090. doi: 10.1126/scitranslmed.abm2090. Epub 2024 Mar 6. PMID: 38446901.
Akihiro Nakamura, Sungsin Jo, Sayaka Nakamura, Ye-Soo Park, Himanshi Gupta, Sandra Vijayan, Jason S. Rockel, Mohit Kapoor, Igor Jurisica, Tae-Hwan Kim, Nigil Haroon. Interacting with MIF enhances neutrophil-driven type 3 immunity and chondrogenesis in murine spondyloarthritis, Cellular & Molecular Immunology, In press.
Go to Catrin home page
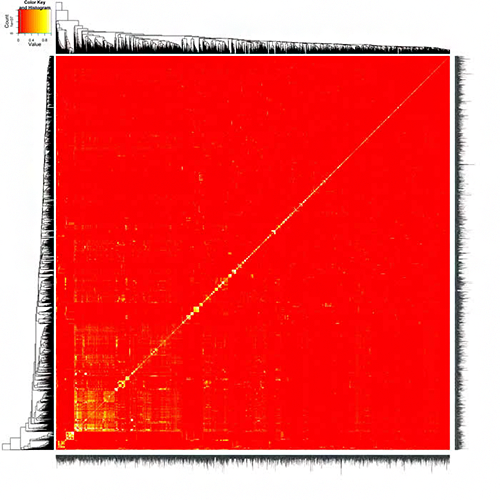
PathDIP 5 provides consolidation for 6,535 human-curated pathways across 12 databases using 7 types and 53 ontological categories. It extends pathway annotation to 3,236 human pathway orphans and includes 5,783 metabolites across species. In addition to enrichment analysis, users can now analyze their results using Drugst.One, where a drug-gene network is created using only the user’s genes in a specific pathway.
Pastrello C, Kotlyar M, Abovsky M, Lu R, Jurisica I. PathDIP 5: improving coverage and making enrichment analysis more biologically meaningful. Nucleic Acids Res, 52(D1):D663-D671, 2024.Pastrello, C, Niu, Y, Jurisica, I. Pathway Enrichment Analysis of Microarray Data. Ed. G. Agapito. Microarray Data Analysis, Methods Mol Biol, vol. 2401: 147-159, 2022.
G. Agapito, Y. Niu, C. Pastrello, I. Jurisica. Pathway integration and annotation: building a puzzle with non-matching pieces and no picture to follow, Briefings in Bioinformatics, 23(5):bbac368, 2022.
Agapito, G., Pastrello, C., Jurisica, I. Comprehensive pathway enrichment analysis workflows: Covid-19 case study, Briefings Bioinform, 22(2): 676-689, 2021.
Rahmati, S., Abovsky, M., Pastrello, C., Kotlyar, M., Lu, R., Cumbaa, C.A., Rahman, P., Chandran, V. and Jurisica, I. pathDIP 4: An extended pathway annotations and enrichment analysis resource for human, model organisms and domesticated species, Nucl Acids Res, 48(D1): D479–D488, 2020.
Agapito G, Pastrello C, Guzzi PH, Jurisica I, Cannataro M. BioPAX-Parser: parsing and enrichment analysis of BioPAX pathways, Bioinformatics, 36(15):4377-4378, 2020.
Rahmati, S., Abovsky, M., Pastrello, C., Jurisica, I. pathDIP: An annotated resource for known and predicted human gene-pathway associations and pathway enrichment analysis. Nucl Acids Res, 45(D1): D419-D426, 2017.
Rahmati, S., Pastrello, C., Rossos, A., Jurisica, I. Two Decades of Biological Pathway Databases: Results and Challenges, In: Ranganathan, S., Nakai, K., Schönbach C. and Gribskov, M. (eds.), Encyclopedia of Bioinformatics and Computational Biology, vol. 1, pp. 1071–1084. Oxford: Elsevier, 2018.
Agapito, G., Pastrello, C., Jurisica, I. Comprehensive pathway enrichment analysis workflows: Covid-19 case study, Briefings Bioinform, 22(2): 676-689, 2021.
Pastrello, C, Niu, Y, Jurisica, I. Pathway Enrichment Analysis of Microarray Data. Ed. G. Agapito. Microarray Data Analysis, Methods Mol Biol, vol. 2401: 147-159, 2022.
Go to pathDIP home page
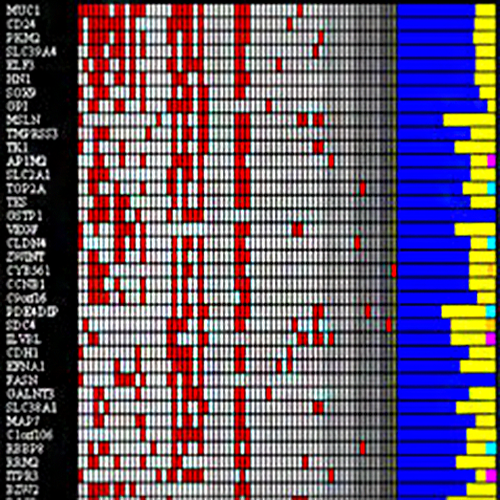
Bhat, M. Elisa Pasini, Chiara Pastrello, Sarah Rahmati, Marc Angeli, Max Kotlyar, Anand Ghanekar and Igor Jurisica, Integrative Analysis of Layers of Data in Hepatocellular Carcinoma Reveals Pathway Dependencies, World J Hepatology, 13(1):94-108, 2021.
Bhat M, Pasini E, Pastrello C, Angeli M, Baciu C, Abovsky M, Coffee A, Adeyi O, Kotlyar M, Jurisica I., Estrogen Receptor 1 Inhibition of Wnt/β-catenin Signaling Contributes to Sex Differences in Hepatocarcinogenesis, Front Oncol. 11:777834, eCollection, 2021
Go to CDIPliver home page
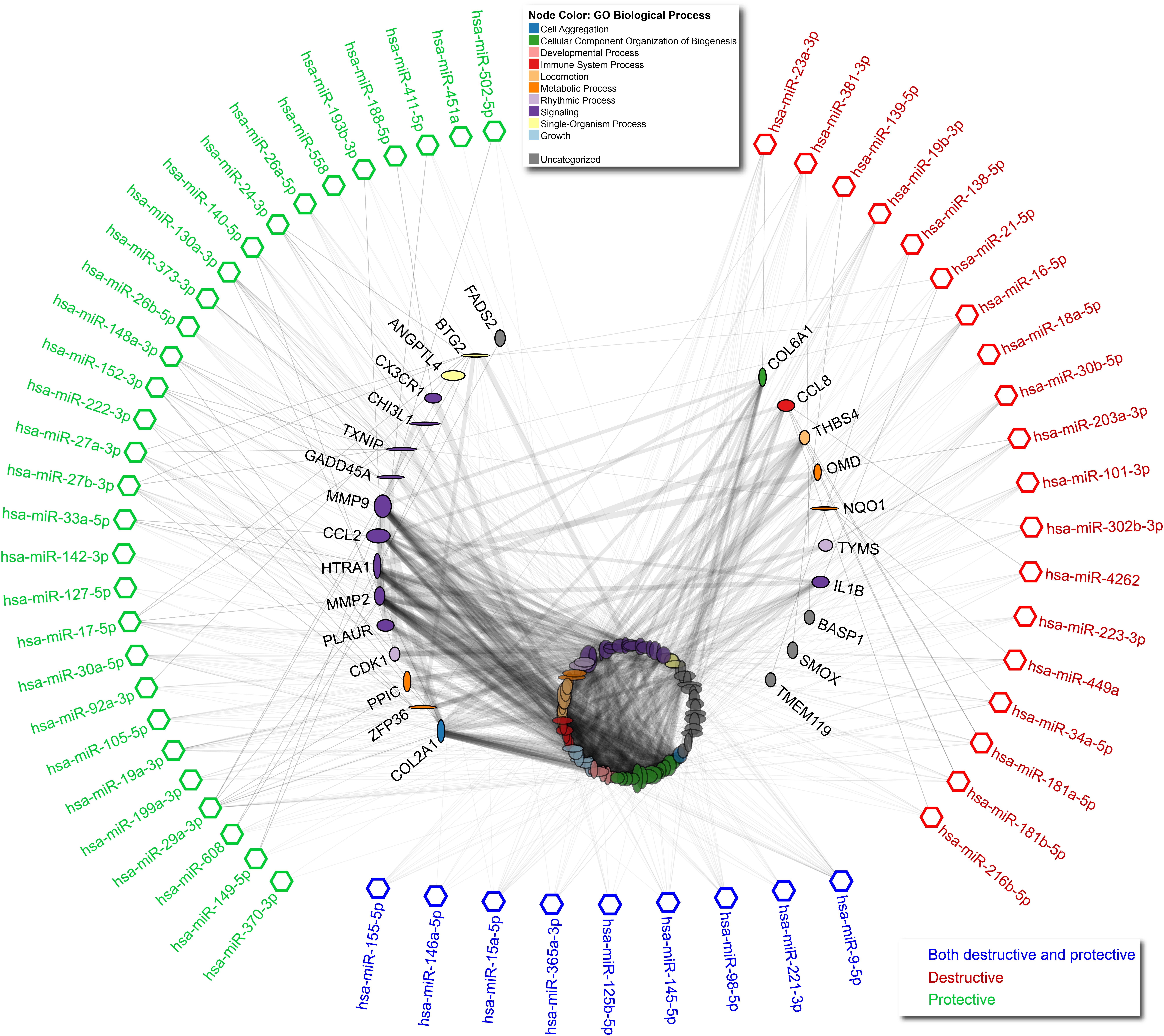
Pastrello C, Abovsky M, Lu R, Ahmed Z, Kotlyar M, Veillette C, Jurisica I, Osteoarthritis Data Integration Portal (OsteoDIP): A web-based gene and non-coding RNA expression database, Osteoarthritis and Cartilage Open, 4(1): 100237, 2022.
Ali SA, Pastrello C, Kaur N, Peffers MJ, Ormseth MJ, Jurisica I. A Network Biology Approach to Understanding the Tissue-Specific Roles of Non-Coding RNAs in Arthritis, Front Endocrinol (Lausanne), 12:744747, eCollection, 2021.
Go to OsteoDIP home page
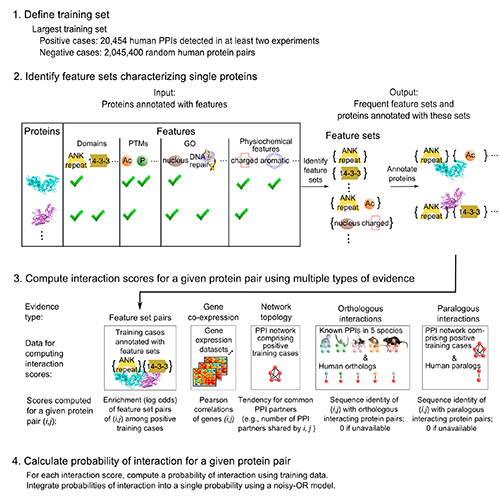
Importantly, we validated 5 of these p53 interactions with orphans by GST pull-down assay (5 of 6 tested -- validation rate of 83%). Overall, validation rates were 40% (2/5) for co-IP, 47% (14/30) for GST pull-down, and 61% (121/198) for MaMTH (Petschnigg et al., Nat Methods, 2014). The high validation rate for MaMTH suggests that FpClass could help guide high-throughput screening, in a combined computational-experimental approach to interactome mapping. This substantially extends our interactome work, including I2D (Brown, Jurisica, Genome Biol, 2007) and (Brown, Jurisica, Bioinformatics, 2005). NAViGaTOR (Brown et al., Bioinformatics, 2009) was used for network analysis and visualization.
Kotlyar M, Pastrello C, Pivetta F, Lo Sardo A, Cumbaa C, Li H, Naranian T, Niu Y, Ding Z, Vafaee F, Broackes-Carter F, Petschnigg J, Mills GB, Jurisicova A, Stagljar I, Maestro R, Jurisica I. In silico prediction of physical protein interactions and characterization of interactome orphans. Nat Methods.12(1):79-847, 2015.
Go to FpClass Supplemental data
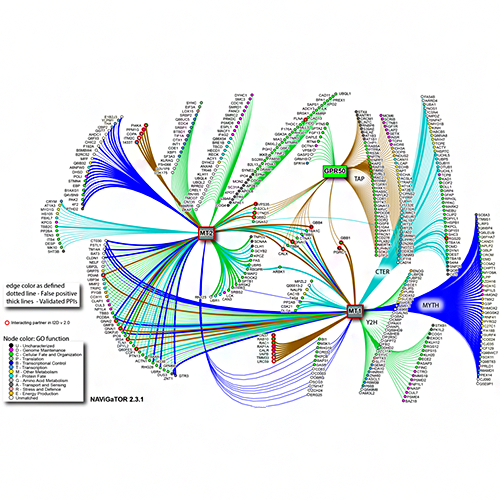
Brown KR, Otasek D, Ali M, McGuffin MJ, Xie W, Devani B, Toch IL, Jurisica I. NAViGaTOR: Network Analysis, Visualization and Graphing Toronto. Bioinformatics. 25(24):3327-9, 2009.
Figure from: Benleulmi-Chaachoua A, Chen L, Sokolina K, Wong V, Jurisica I, Emerit MB, Darmon M, Espin A, Stagljar I, Tafelmeyer P, Zamponi GW, Delagrange P, Maurice P, Jockers R. Protein interactome mining defines melatonin MT1 receptors as integral component of presynaptic protein complexes of neurons. J Pineal Res. 60(1):95-108, 2016.
Hauschild, AC, Pastrello, C., Rossos, A., Jurisica, I. Visualization of Biomedical Networks, In: Ranganathan, S., Nakai, K., Schönbach C. and Gribskov, M. (eds.), Encyclopedia of Bioinformatics and Computational Biology, vol. 1, pp. 1016–1035. Oxford: Elsevier, 2018.
Go to NAViGaTOR home page
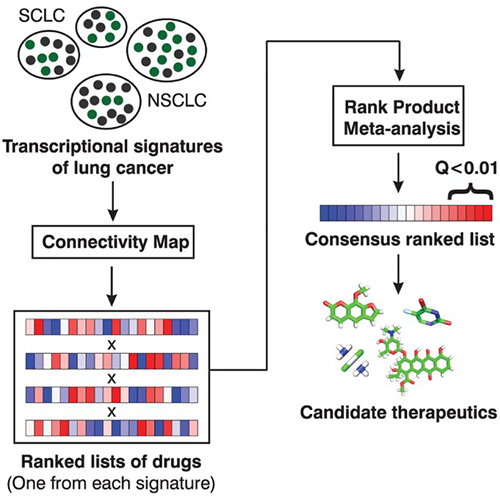
Fortney K, Griesman J, Kotlyar M, Pastrello C, Angeli M, Sound-Tsao M, Jurisica I. Prioritizing therapeutics for lung cancer: an integrative meta-analysis of cancer gene signatures and chemogenomic data. PLoS Comput Biol. 11(3):e1004068, 2015.
Go to CMapBatch home page
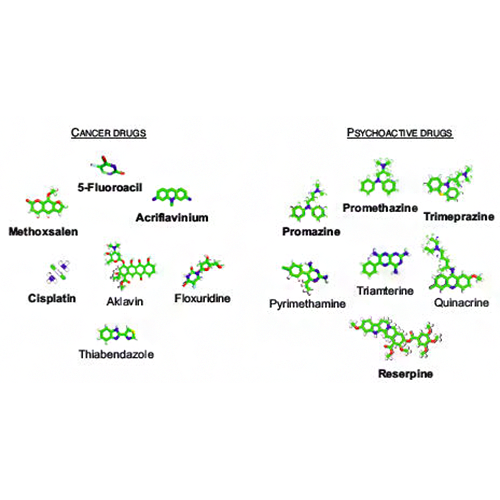
Fortney K, Xie W, Kotlyar M, Griesman J, Kotseruba Y, Jurisica I. NetwoRx: connecting drugs to networks and phenotypes in Saccharomyces cerevisiae. Nucleic Acids Res. 41:D720-7, 2013
Go to NetwoRx home page
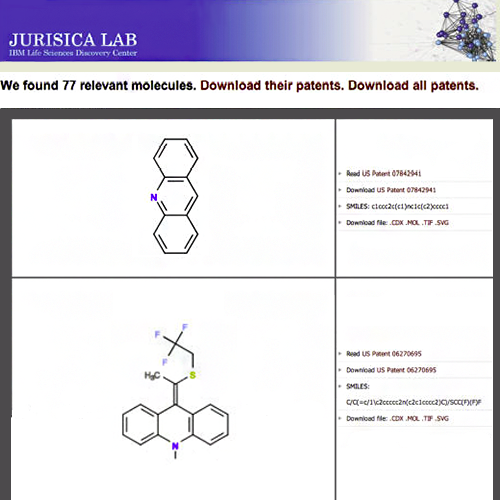
Heifets A, Jurisica I. SCRIPDB: a portal for easy access to syntheses, chemicals and reactions in patents. Nucleic Acids Res. 40:D428-33, 2012
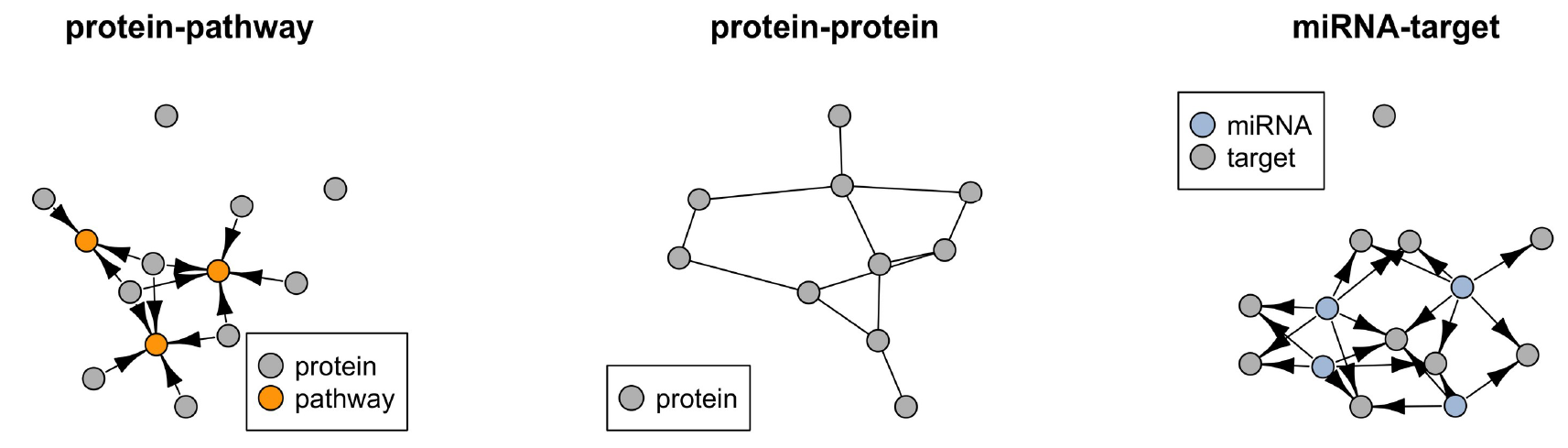
Tomas Tokar, Chiara Pastrello, Mark Abovsky, Sara Rahmati, Igor Jurisica, miRAnno—network-based functional microRNA annotation, Bioinformatics, 38(2):592–593, 2022.
Go to miRAnno home page
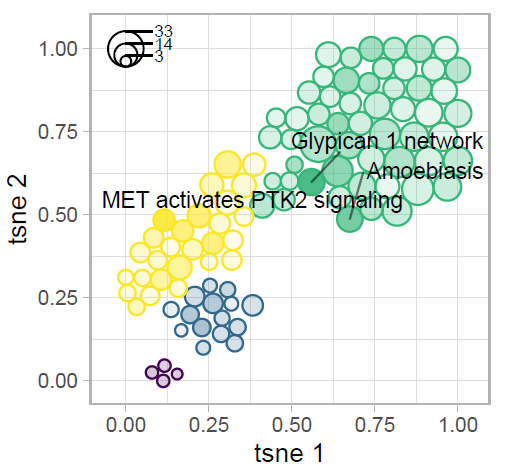
Tokar, T., Pastrello, C., Jurisica, I. GSOAP: A tool for visualization of gene set over-representation analysis, Bioinformatics, 36(9):2923-2925, 2020.
Go to GSOAP home page
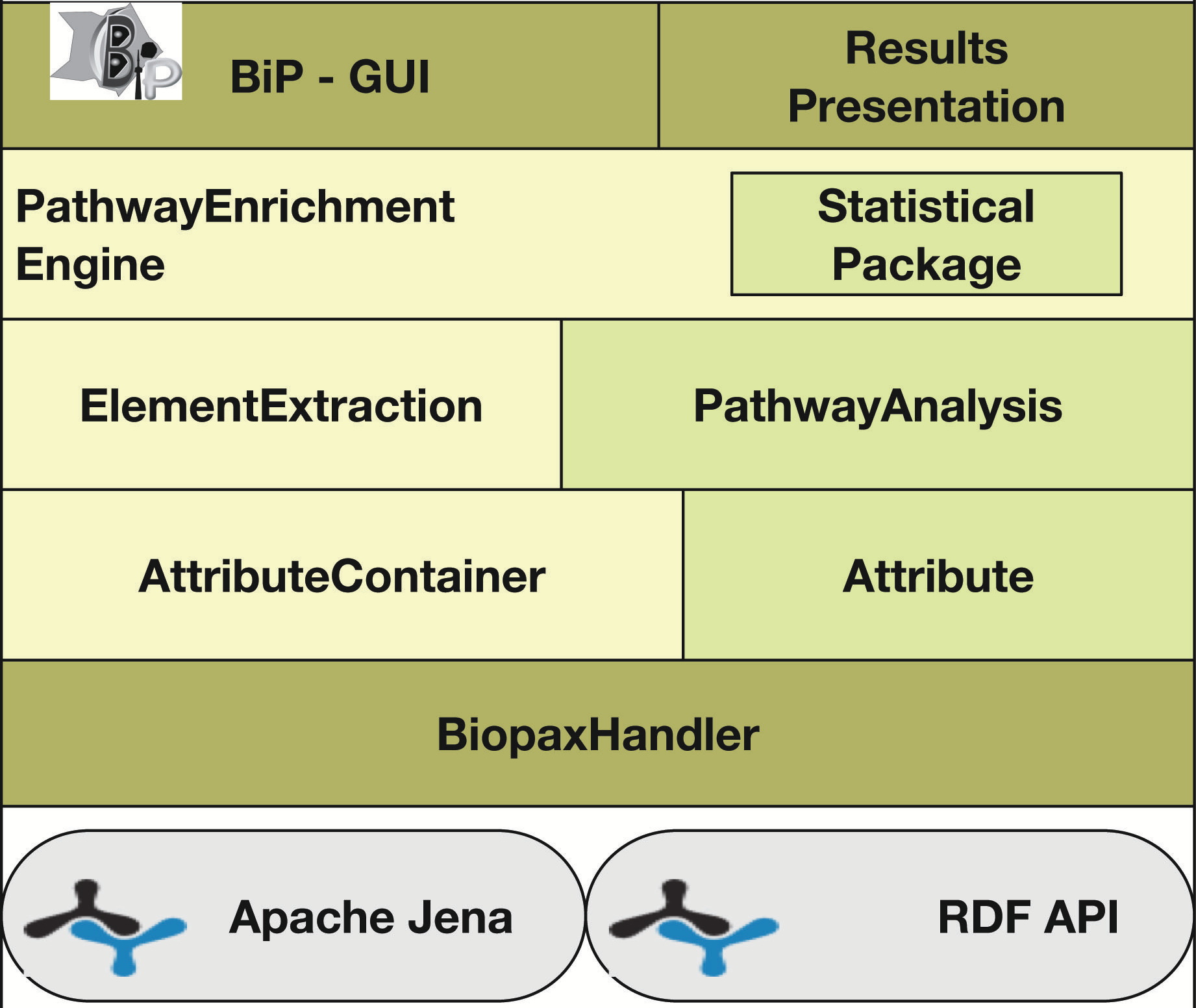
Agapito G, Pastrello C, Guzzi PH, Jurisica I, Cannataro M. BioPAX-Parser: parsing and enrichment analysis of BioPAX pathways, Bioinformatics, 36(15):4377-4378, 2020.
Go to BIP home page
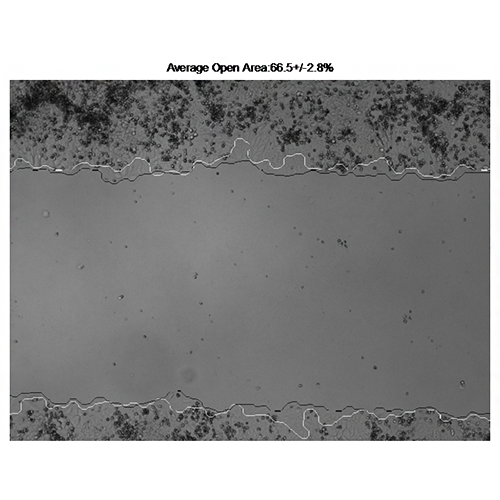
Vargas A, Angeli M, Pastrello C, McQuaid R, Li H, Jurisicova A, Jurisica I. Robust quantitative scratch assay. Bioinformatics. 32(9):1439-40, 2016
Go to RQSA home page
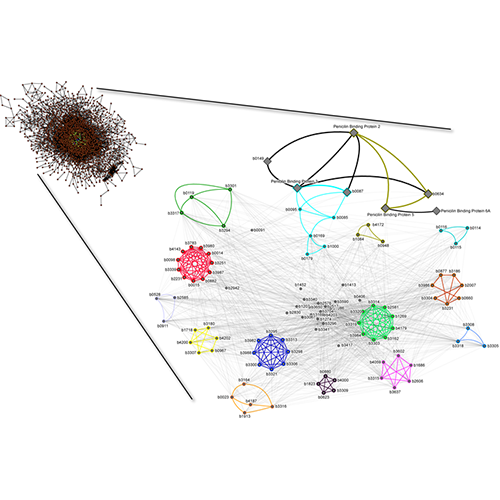
King AD, Przulj N, Jurisica I. Protein complex prediction via cost-based clustering. Bioinformatics. 20(17):3013-20, 2004.
Go to RNSC home page
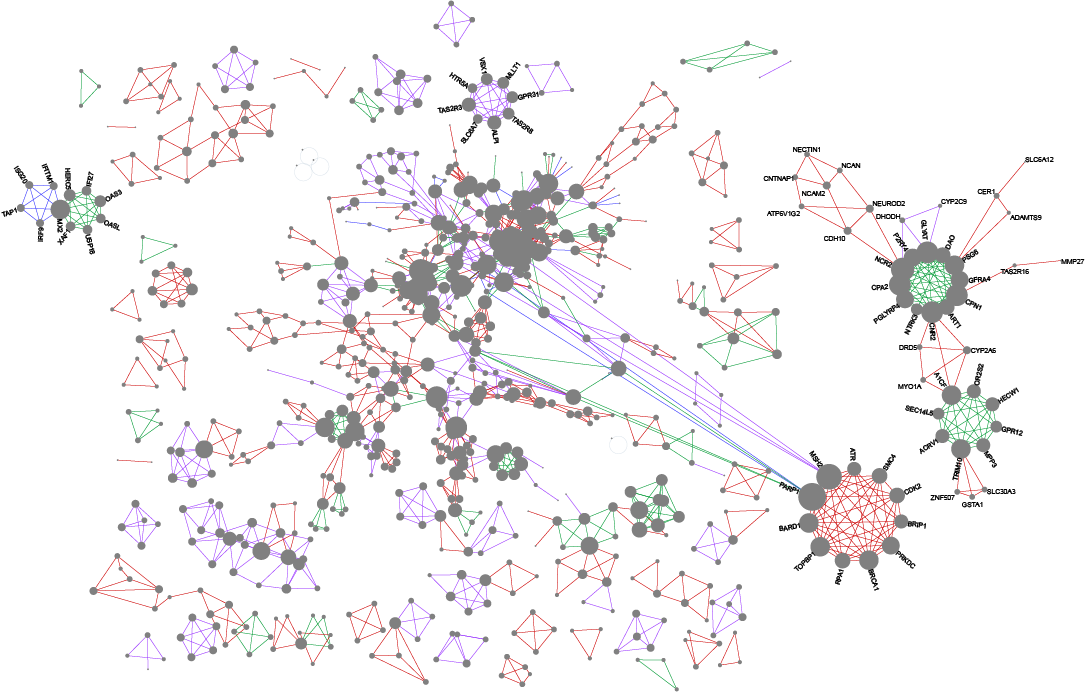
Wong SWH, Pastrello C, Kotlyar M, Faloutsos C, Jurisica I. USNAP: Fast unique dense region detection and its application to lung cancer, Bioinformatics, 39(8):btad477, 2023
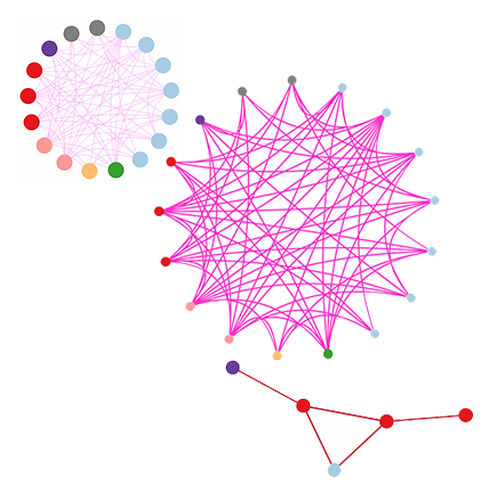
Wong, S., Pastrello, C., Kotlyar, M., Faloutsos, C., Jurisica, I. SDREGION: Fast spotting of changing communies in biological networks. Proceedings of the ACM SIGKDD International Conference on Knowledge Discovery and Data Mining, pp. 867-875, 2018.
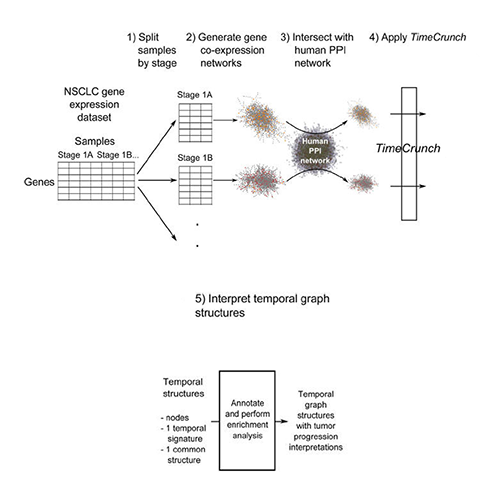
Wong SWH, Pastrello C, Kotlyar M, Faloutsos C, Jurisica I. Modeling tumor progression via the comparison of stage-specific graphs. Methods. 2018 Jan 1;132:34-41. doi: 10.1016/j.ymeth.2017.06.033. Epub 2017 Jul 3. PubMed PMID: 28684340.
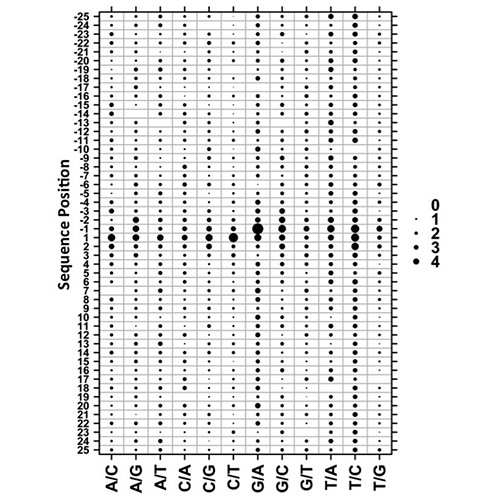
Yan, R., Boutros, P.C., Jurisica, I. A tree-based approach for motif discovery and sequence classification. Bioinformatics. 27(15):2054-61, 2011.
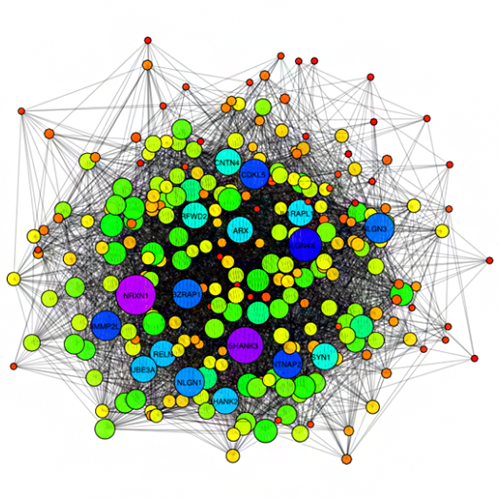
Vafaee F, Rosu D, Broackes-Carter F, Jurisica I. Novel semantic similarity measure improves an integrative approach to predicting gene functional associations. BMC Syst Biol. 7:22, 2013.
Go to GAP home page
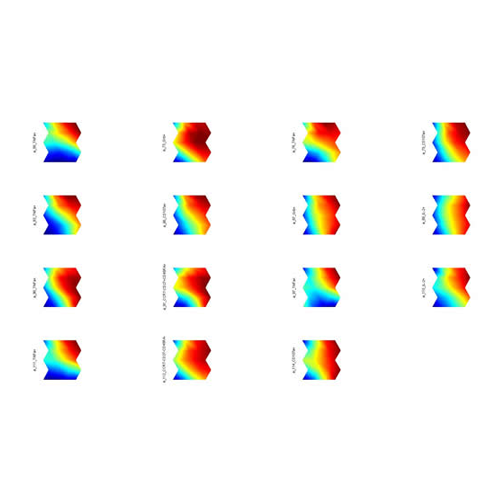
Sultan M, Wigle DA, Cumbaa CA, Maziarz M, Glasgow J, Tsao MS, Jurisica I. Binary tree-structured vector quantization approach to clustering and visualizing microarray data. Bioinformatics. 18 Suppl 1:S111-9, 2002.
Go to BTSVQ home page