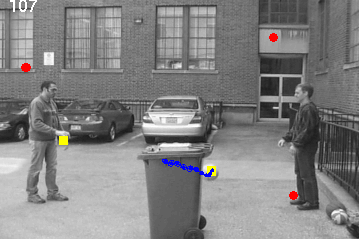
Introduction
We propose a new model for the probabilistic estimation of continuous state variables from a sequence of observations, such as tracking the position of an object in video. This mapping is modeled as a product of dynamics experts (features relating the state at adjacent time-steps) and observation experts (features relating the state to the image sequence). Individual features are flexible in that they can switch on or off at each time-step depending on their inferred relevance (or on additional side information), and discriminative in that they need not model the full generative likelihood of the data. When trained conditionally, this permits the inclusion of a broad range of rich features (for example, features relying on observations from multiple time-steps), and allows the relevance of features to be learned from labeled sequences.
Publications
- Combining Discriminative Features to Infer Complex
Trajectories
David Ross, Simon Osindero, and Richard Zemel. In Proceedings of the Twenty-Third International Conference on Machine Learning, 2006. [PS.GZ] [PDF]
Videos
Here are the results of applying our model to the problem of tracking a basketball. The etimated location of ball is given in blue, with the size of the ellipse indicating the uncertainty in position. The predicted locations of the basketball, according to each of the individual observation features, are given by yellow boxes (if our model turns the feature on during that timestep) and red circles (if the feature is turned off).
- Simon sequence
In this experiment we used the first 500 frames, hand-labeled with the location of the basketball, to train the model, then tested on the remaining frames. Included in the video are results on the training and testing frames--results on the testing frames alone are indistinguishable.
- Rolling+Bouncing sequences
Here the model was trained on the first 500 frames of the rolling sequence and the bouncing sequence, then tested on the remaining frames, as well as on a previously-unseen sequence containing both rolling and bouncing.
- Total occlusion
The model from above, trained on rolling and bouncing, applied to a new sequence in which the ball completely disappears behind a bin:
- Trouble viewing the videos? Please email me if there are any problems viewing the videos. The VLC media player should be able to show them on all platforms. On a Mac, installing Perian will let you view them in Quicktime.
Code
- Matlab code which implements this learning/tracking algorithm is available here: cdf_2007-07-13.zip.
- Raw video data from these sequences is available, along with the corresponding ground truth locations of the basketball. Please email me if interested.
Presentations
- Google Tech Talk (June 2006): view it online
- ICML 2006 Talk: slides are available here [PDF]
- ML Group Meeting Talk
These are the slides from my talk at the Toronto Machine Learning group meeting on Feb. 6, 2006.[PDF]