Does semaglutide protect kidney health? Do later school times promote the well-being of children? These types of questions about cause and effect drive decisions across medicine, policy, and business. Randomized controlled trials (RCTs) are the most trusted mechanism to answer causal questions by estimating treatment effects. Unfortunately, clinical trials take several years and millions of dollars to maybe approve a drug, and RCTs are often infeasible for many critical policy questions. Yet, a sudden outbreak or pandemic gives us mere days and a handful of potential facts to make vital decisions. Our aim is to bolster the sources of causal information available to us and accelerate the extraction of useful insights from them.
Observational studies offer pre-trial insights but demand structured data. Meanwhile, a wealth of information lies untapped in online forums. For instance, thousands with diabetes, migraines, or Long Covid share their treatment experiences on dedicated subreddits. This data is rich, diverse, and accessible – but unstructured. In this work, we introduce a pipeline that turns unstructured text data like this into causal insights.
NATURAL: From Forums to Findings
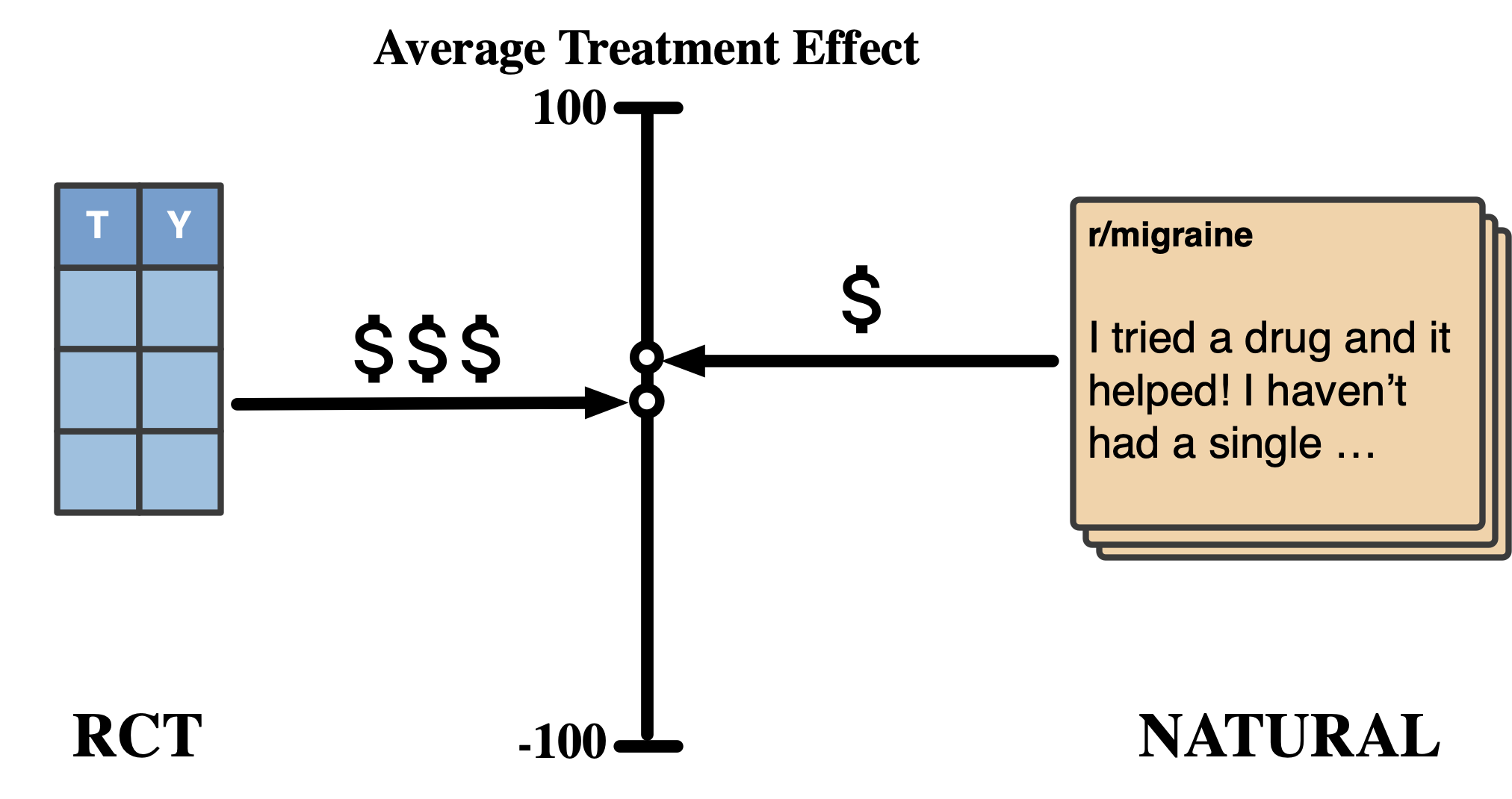
unstructured text data from online forums to cheap ATE estimates in hours.
NATURAL is a large-language-model based pipeline that turns unstructured text data into meaningful treatment effects. We used social media data to test its performance against real-world RCTs comparing several diabetes and migraine drugs. For clinical trials, NATURAL predicted average treatment effects (ATEs) that fell within three percentage points of their ground truth counterparts! This suggests that unstructured text data is indeed a rich source of causal effect information, and NATURAL is a first step towards an automated pipeline to tap this resource.
Semaglutide vs. Tirzepatide NCT03987919 |
Semaglutide vs. Liraglutide NCT03191396 |
Erenumab vs. Topiramate NCT03828539 |
OnabotulinumtoxinA vs. Topiramate NCT02191579 |
|
---|---|---|---|---|
Treatment effect in real-world RCT |
10.11 | -14.70 | 28.30 | 41.00 |
NATURAL using social media data |
9.06 | -16.57 | 29.05 | 42.53 |
How It Works
NATURAL is data-driven, but also incorporates domain expertise. At a high-level, the key steps to compute NATURAL estimators are:- Domain expertise is used to design an observational study, ensuring it meets critical causal assumptions.
- A multi-step filtering identifies reports that are likely to conform to the experimental design.
- Large language models (LLMs) are used to extract the conditional distribution of structured variables of interest (outcome, treatment, covariates) given the report.
- We adapt classical techniques like inverse propensity score weighting to compute treatment effect estimates from these conditionals.
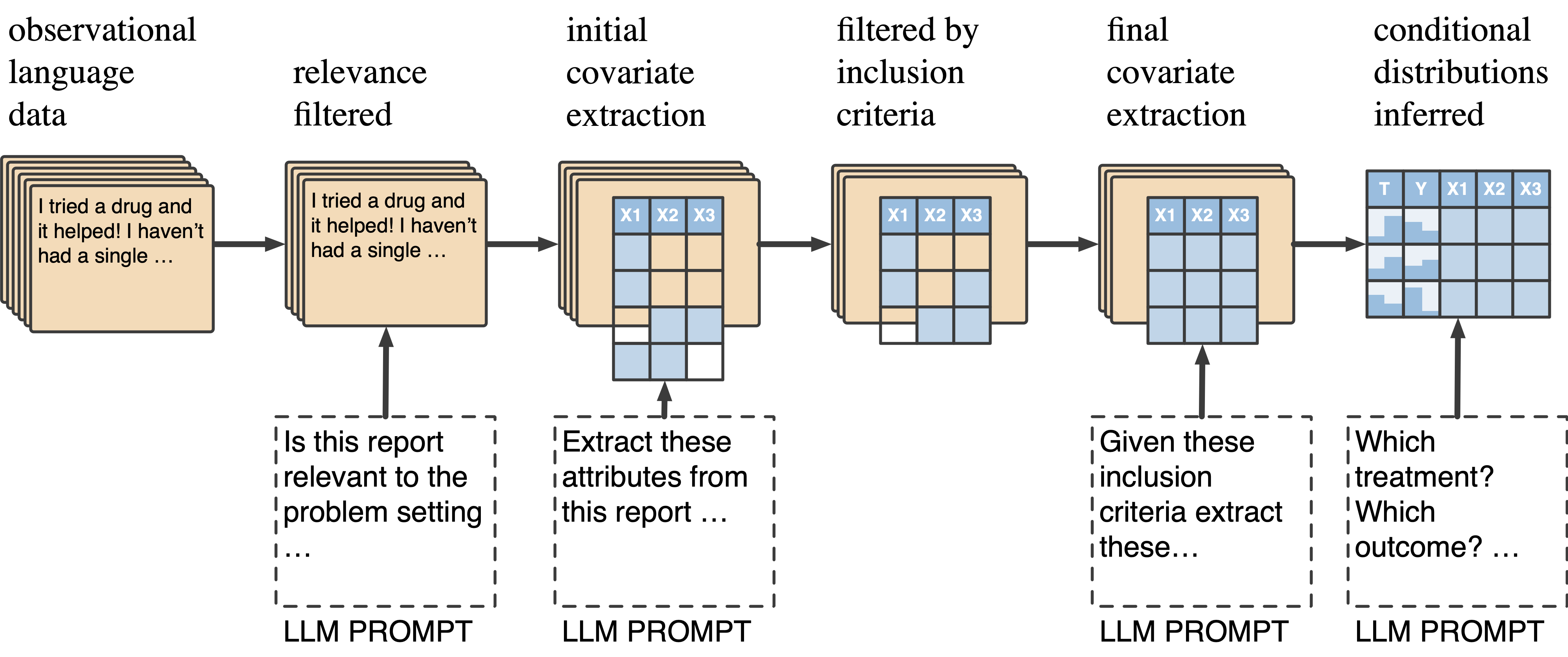
Evaluation
We developed six observational datasets to evaluate different parts of NATURAL: two synthetic datasets constructed using marketing data, and four clinical datasets curated from public (pre-December 2022) migraine and diabetes subreddits from the Pushshift collection. For each dataset, we treated the average treatment effect (ATE) from a corresponding real-world completely randomized experiment as ground truth. You can see the results obtained with our NATURAL estimators in the table above. In synthetic settings, we can conduct more fine-grained evaluation than simply comparing the final ATEs. The visualization below suggests that LLMs are able to estimate observational distributions increasingly well with self-reported data.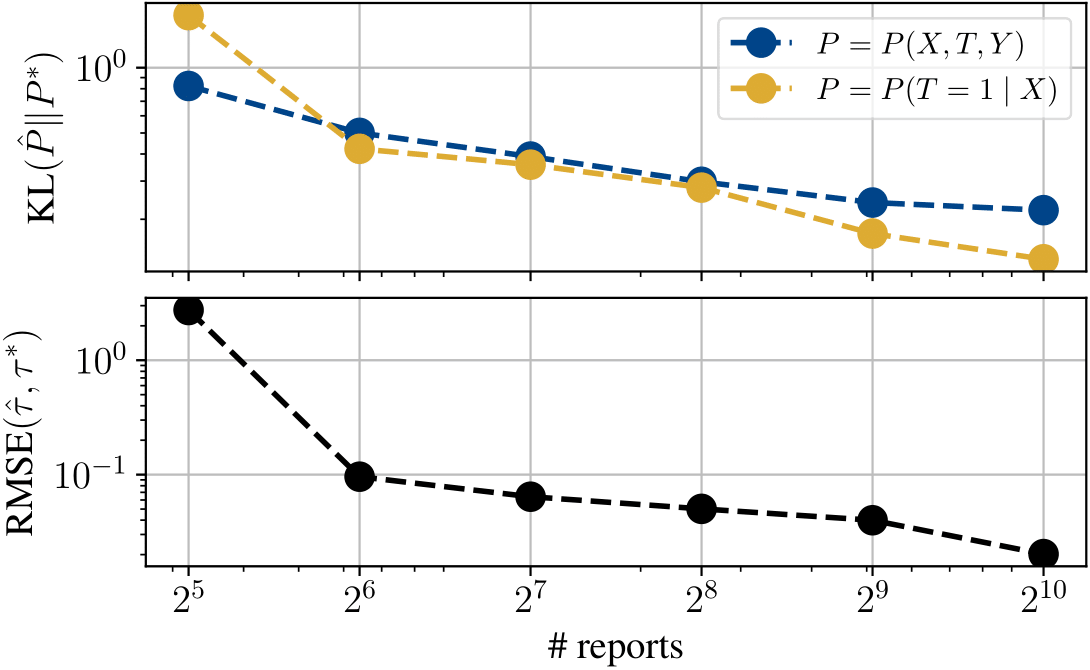
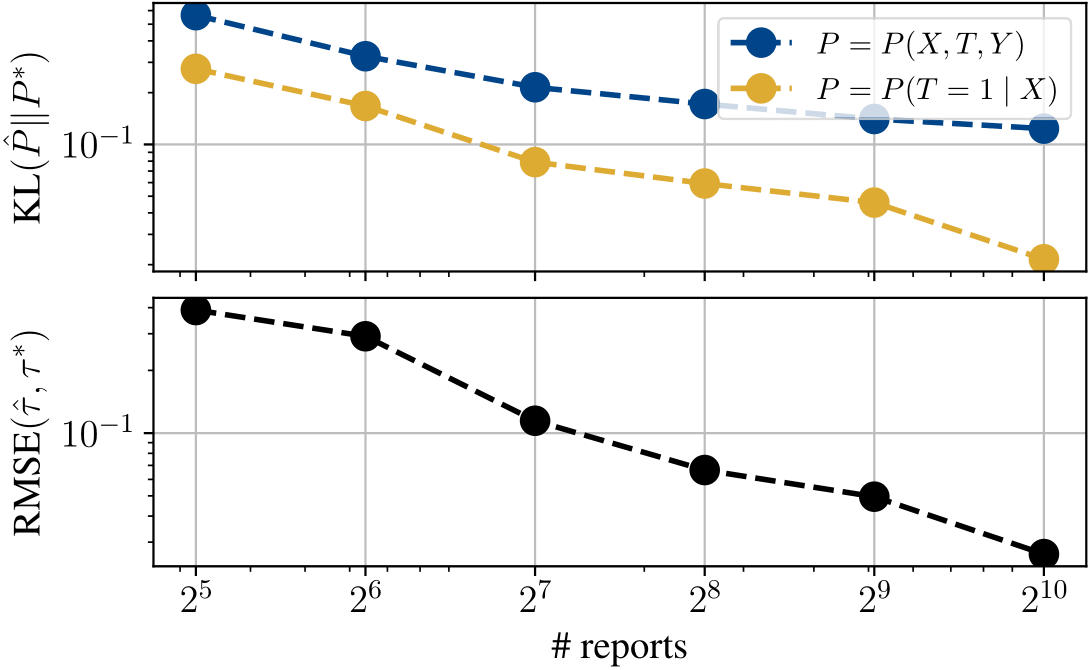
counterparts reduces with increasing number of posts (top), as does the RMSE between the NATURAL estimate and true ATE (bottom).
What's Next
NATURAL is a first step towards automated effect estimation from natural language data, with further potential in data-driven decision-making. We are excited to expand its data sources as well as applications:- Using online forum conversations to better understand policy interventions.
- Estimating individualized treatment effects on-demand from electronic health records.
- Prioritizing clinical trial investment for neglected diseases, based on real lived experiences.
- Repurposing drugs and uncovering hidden potential in existing medications.
- Detecting rare adverse effects of drugs via safety monitoring in large, diverse populations.