 |
 |
 |
 |
 |
 |
 |
 |
|
|
Why does greedy learning work?
|
|
|
|
|
 |
 |
The weights,
W, in the bottom level RBM define
|
p(v|h) and they
also, indirectly, define p(h).
|
|
|
So we can express the RBM
model as
|
|
|
|
|
|
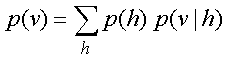 |
|
|
|
 |
 |
 |
 |
If we leave
p(v|h) alone and build a better model of
|
|
p(h), we will
improve p(v).
|
|
|
We need a better
model of the aggregated
posterior
|
distribution over
hidden vectors produced by
|
|
applying W to the data.
|
|
|
|
|