Abstract
Deep learning methods are powerful tools but often suffer from expensive computation and limited flexibility. An alternative is to combine light-weight models with deep representations. As successful cases exist in several visual problems, a unified framework is absent. In this paper, we revisit two widely used approaches in computer vision, namely filtered channel features and Convolutional Neural Networks (CNN), and absorb merits from both by proposing an integrated method called Convolutional Channel Features (CCF). CCF transfers low-level features from pre-trained CNN models to feed the boosting forest model. With the combination of CNN features and boosting forest, CCF benefits from the richer capacity in feature representation compared with channel features, as well as lower cost in computation and storage compared with end-to-end CNN methods. We show that CCF serves as a good way of tailoring pre-trained CNN models to diverse tasks without fine-tuning the whole network to each task by achieving state-of-the-art performances in pedestrian detection, face detection, edge detection and object proposal generation.Approach
Results
1. Pedestrian detection on Caltech (a more detailed comparison)
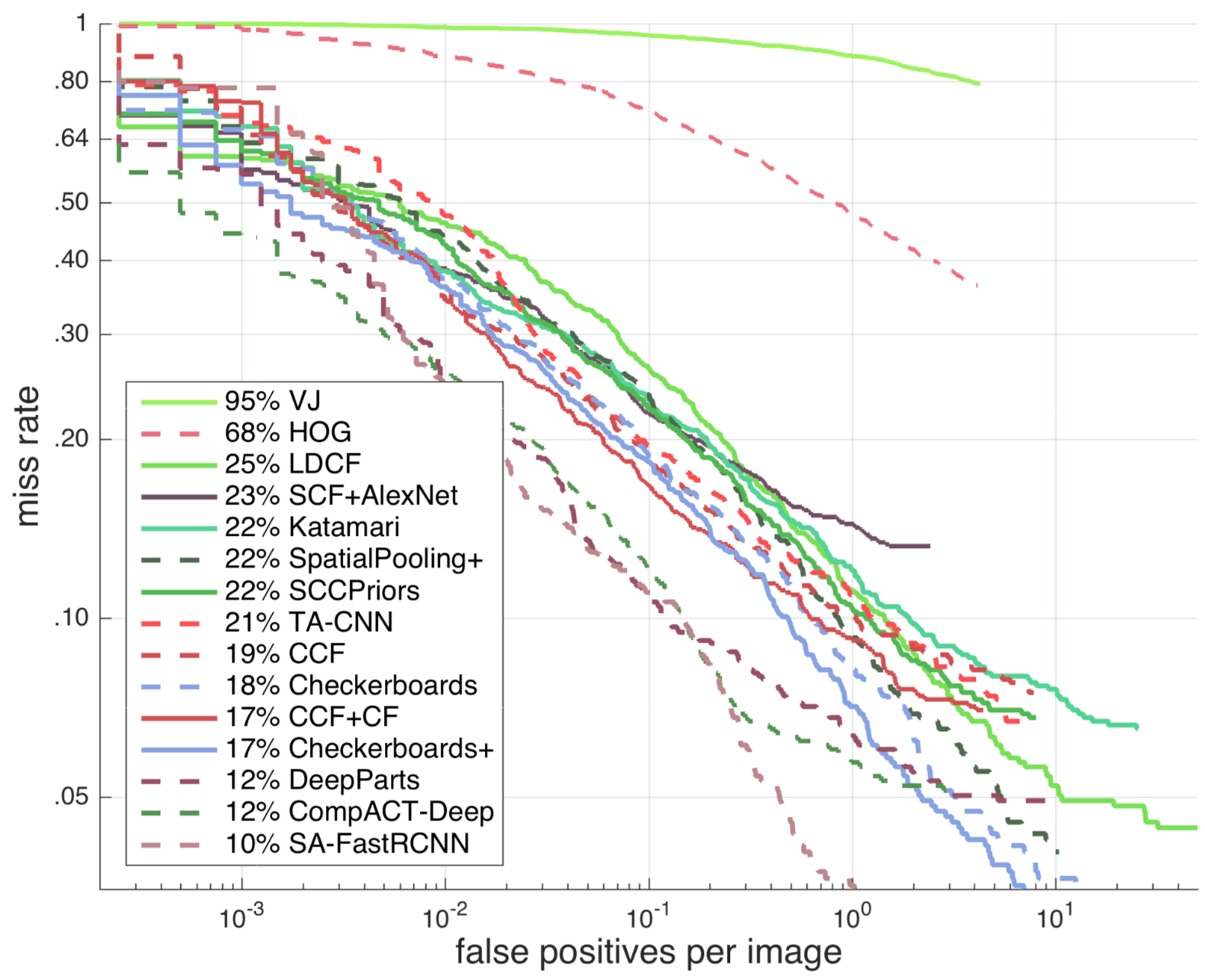
2. Face detection on AFW and FDDB
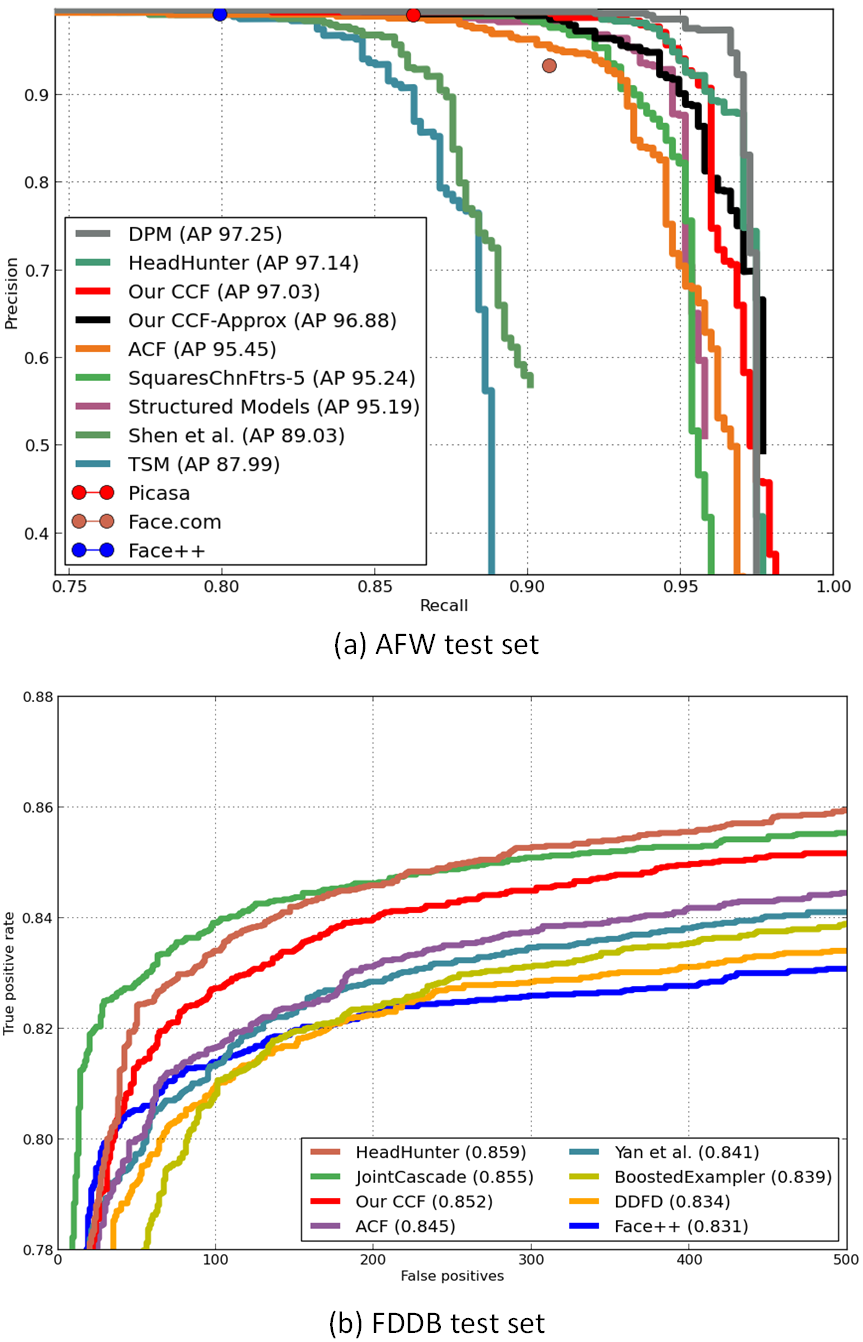
3. Edge detection on BSDS500
Evaluation result of edge detection on BSDS500 dataset. Three standard metrics are used, which are fixed contour threshold (ODS), per-image best threshold (OIS), and average precision (AP).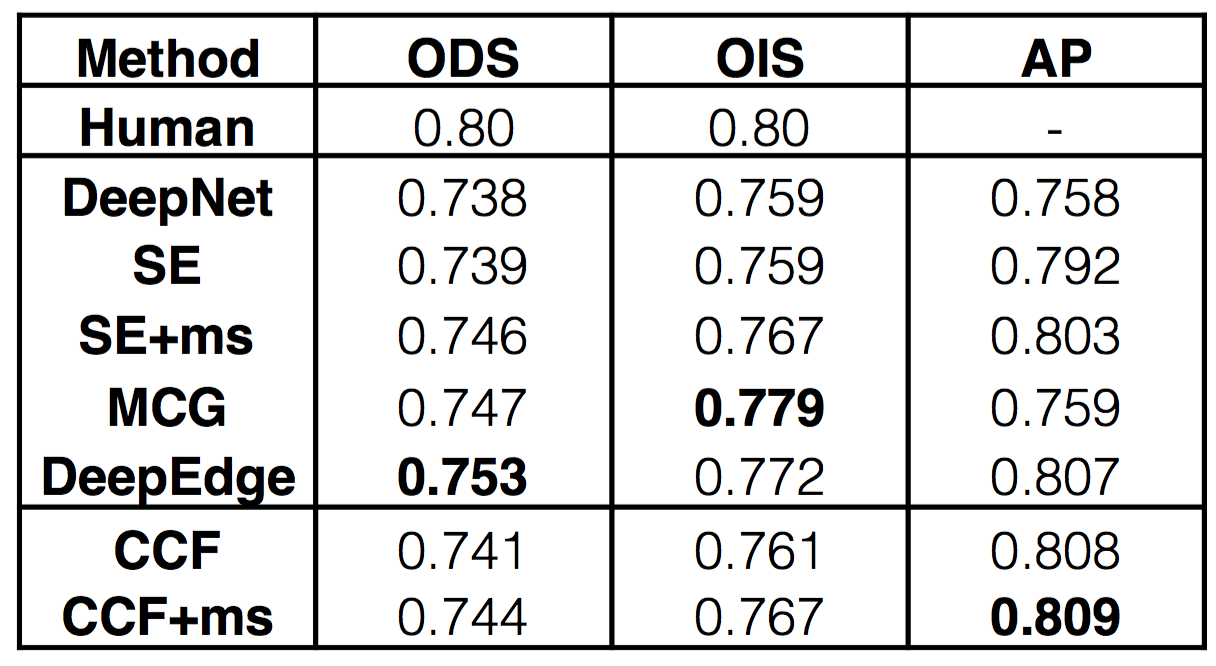
4. Object proposal generation on PASCAL VOC 2007
Evaluation result of object proposal generation on PASCAL VOC 2007 test set with IoU threshold of 0.7. Metrics are Area Under Curve (AUC), number of proposals needed to reach 50% and 75% recall rate and maximum recall rate.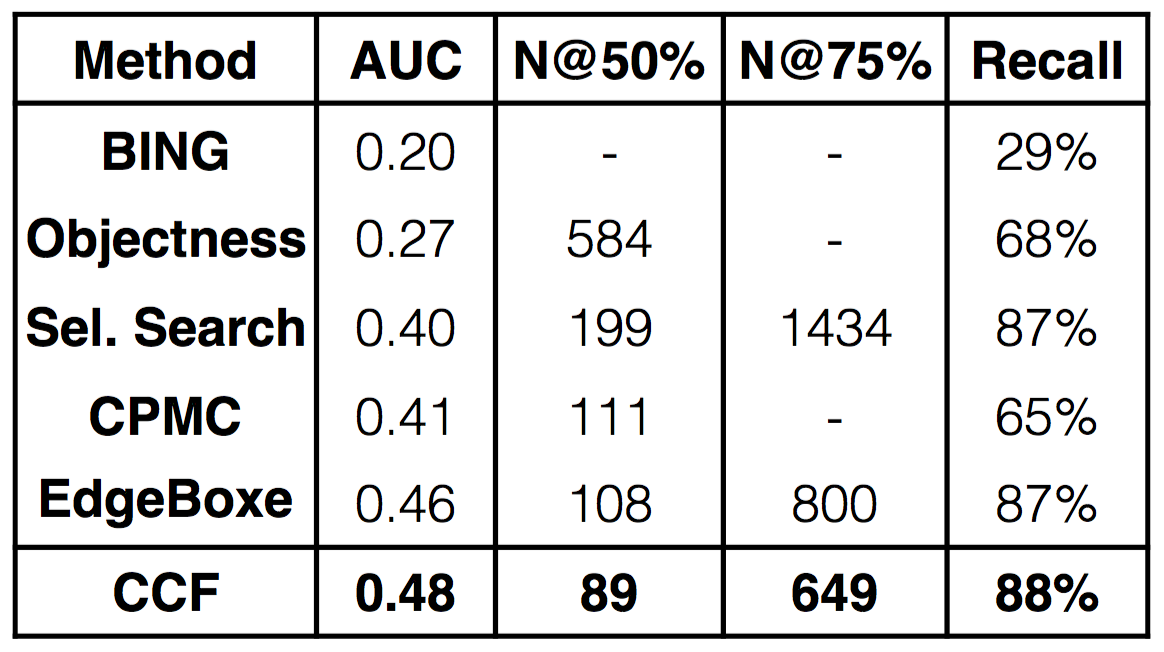
Download
2. Code for pedestrian detection
3. Curve data on Caltech (CCF+CF, CCF), AFW (using Mathias's protocol), FDDB (DiscROC, ContROC)
BibTeX
@inproceedings{binyang15ccf, Author = {Bin Yang and Junjie Yan and Zhen Lei and Stan Z. Li}, Title = {Convolutional Channel Features}, Booktitle = {Proceedings of the IEEE International Conference on Computer Vision (ICCV)}, Year = {2015} }