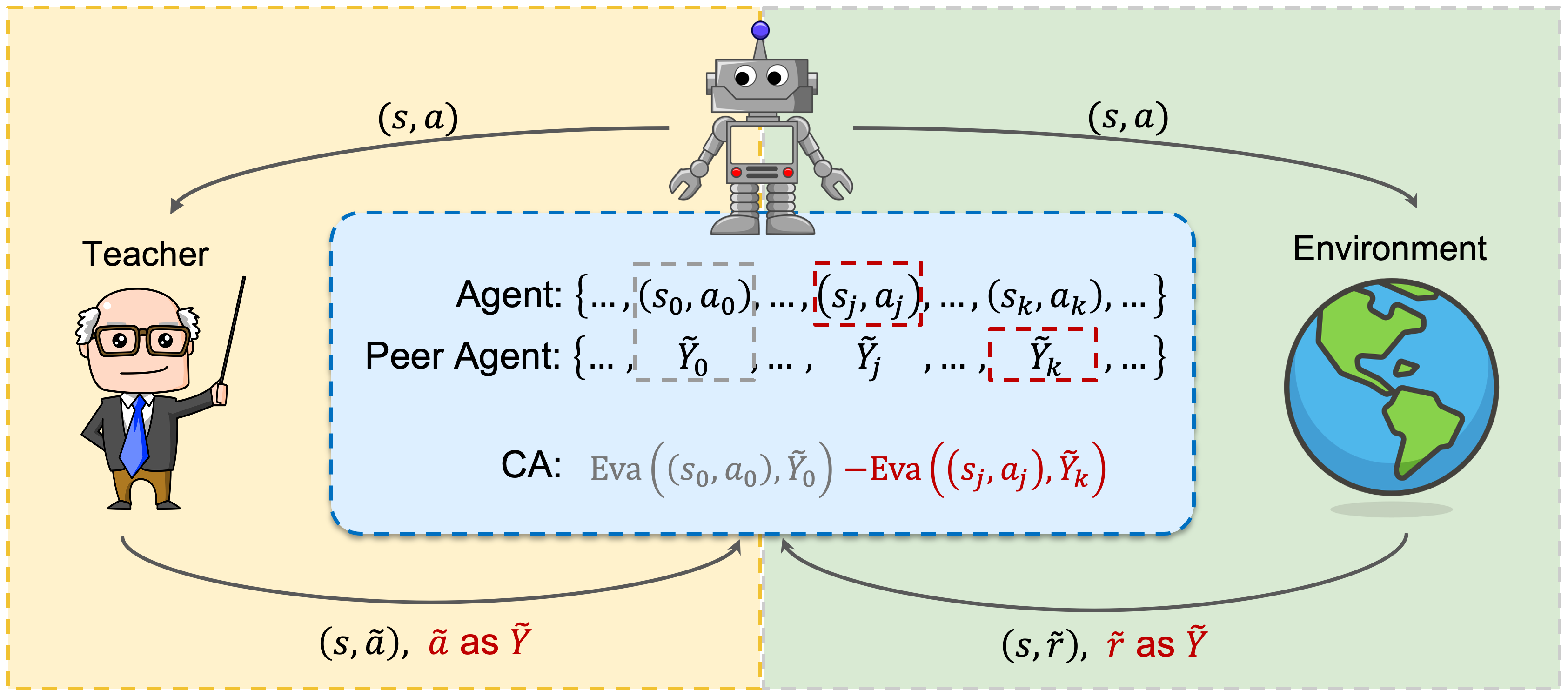
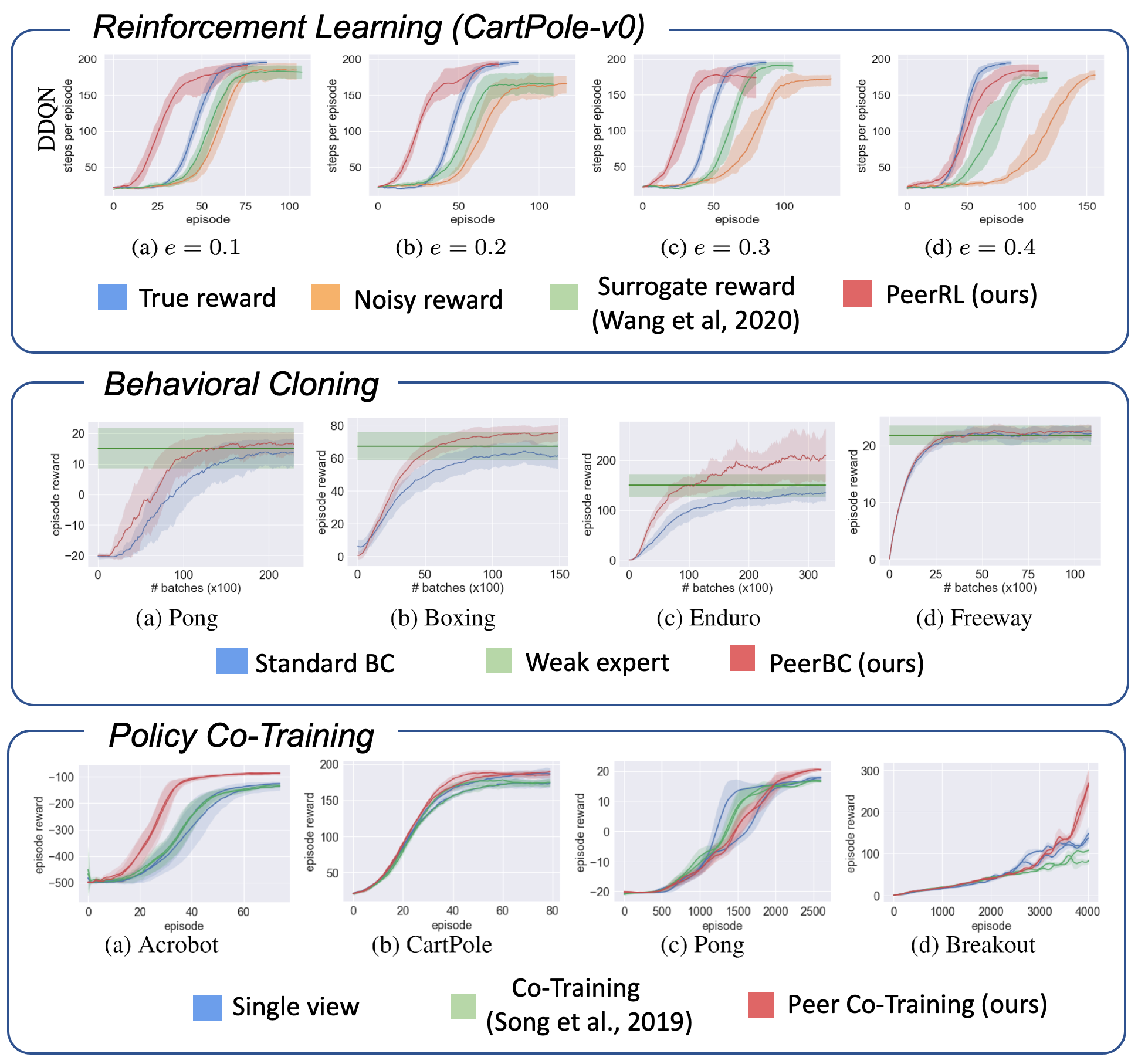
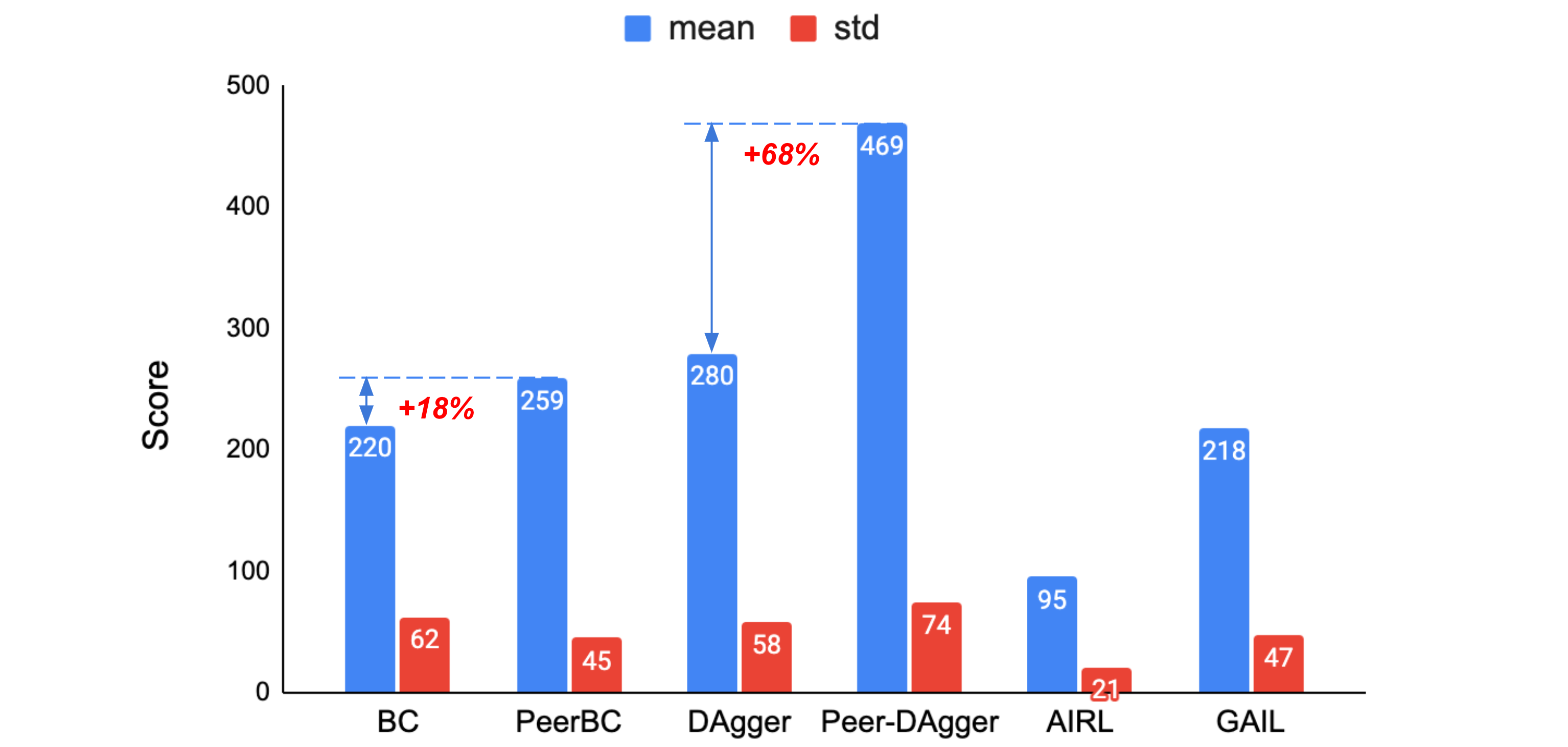
BibTeX
@inproceedings{wang2021policy, title = {Policy Learning Using Weak Supervision}, author = {Jingkang Wang and Hongyi Guo and Zhaowei Zhu and Yang Liu}, booktitle = {Thirty-Fifth Conference on Neural Information Processing Systems}, year = {2021}, url = {https://openreview.net/forum?id=UZgQhsTYe3R} }
Text citation
Jingkang Wang, Hongyi Guo, Zhaowei Zhu and Yang Liu. Policy Learning Using Weak Supervision. In Thirty-Fifth Conference on Neural Information Processing Systems (NeurIPS), 2021.
@inproceedings{liu2020peer, title = {Peer Loss Functions: Learning from Noisy Labels without Knowing Noise Rates}, author = {Yang Liu and Hongyi Guo}, booktitle = {Thirty-Seventh International Conference on Machine Learning}, year = {2020}, }
@inproceedings{wang2020reinforcement, title = {Reinforcement Learning with Perturbed Rewards}, author = {Jingkang Wang and Yang Liu and Bo Li}, booktitle = {Thirty-Fourth AAAI Conference on Artificial Intelligence}, year = {2020}, }